LAPSE:2023.8650
Published Article
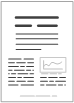
LAPSE:2023.8650
Feasibility Study on the Influence of Data Partition Strategies on Ensemble Deep Learning: The Case of Forecasting Power Generation in South Korea
February 24, 2023
Ensemble deep learning methods have demonstrated significant improvements in forecasting the solar panel power generation using historical time-series data. Although many studies have used ensemble deep learning methods with various data partitioning strategies, most have only focused on improving the predictive methods by associating several different models or combining hyperparameters and interactions. In this study, we contend that we can enhance the precision of power generation forecasting by identifying a suitable data partition strategy and establishing the ideal number of partitions and subset sizes. Thus, we propose a feasibility study of the influence of data partition strategies on ensemble deep learning. We selected five time-series data partitioning strategies—window, shuffle, pyramid, vertical, and seasonal—that allow us to identify different characteristics and features in the time-series data. We conducted various experiments on two sources of solar panel datasets collected in Seoul and Gyeongju, South Korea. Additionally, LSTM-based bagging ensemble models were applied to combine the advantages of several single LSTM models. The experimental results reveal that the data partition strategies positively influence the forecasting of power generation. Specifically, the results demonstrate that ensemble models with data partition strategies outperform single LSTM models by approximately 4−11% in terms of the coefficient of determination (R2) score.
Record ID
Keywords
data partition, long short-term memory, power generation, Solar Panels, solar panels with weather
Subject
Suggested Citation
Chuluunsaikhan T, Kim JH, Shin Y, Choi S, Nasridinov A. Feasibility Study on the Influence of Data Partition Strategies on Ensemble Deep Learning: The Case of Forecasting Power Generation in South Korea. (2023). LAPSE:2023.8650
Author Affiliations
Chuluunsaikhan T: Department of Computer Science, Chungbuk National University, Cheongju 28644, Korea [ORCID]
Kim JH: Department of Computer Science, Chungbuk National University, Cheongju 28644, Korea
Shin Y: Department of Computer Science, Chungbuk National University, Cheongju 28644, Korea
Choi S: Department of Management Information Systems, Chungbuk National University, Cheongju 28644, Korea; Department of Bigdata, Chungbuk National University, Cheongju 28644, Korea
Nasridinov A: Department of Computer Science, Chungbuk National University, Cheongju 28644, Korea
Kim JH: Department of Computer Science, Chungbuk National University, Cheongju 28644, Korea
Shin Y: Department of Computer Science, Chungbuk National University, Cheongju 28644, Korea
Choi S: Department of Management Information Systems, Chungbuk National University, Cheongju 28644, Korea; Department of Bigdata, Chungbuk National University, Cheongju 28644, Korea
Nasridinov A: Department of Computer Science, Chungbuk National University, Cheongju 28644, Korea
Journal Name
Energies
Volume
15
Issue
20
First Page
7482
Year
2022
Publication Date
2022-10-11
Published Version
ISSN
1996-1073
Version Comments
Original Submission
Other Meta
PII: en15207482, Publication Type: Journal Article
Record Map
Published Article
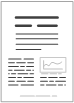
LAPSE:2023.8650
This Record
External Link
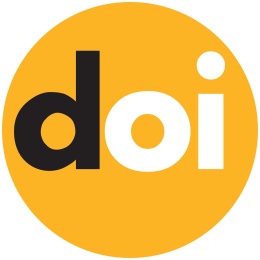
doi:10.3390/en15207482
Publisher Version
Download
Meta
Record Statistics
Record Views
107
Version History
[v1] (Original Submission)
Feb 24, 2023
Verified by curator on
Feb 24, 2023
This Version Number
v1
Citations
Most Recent
This Version
URL Here
https://psecommunity.org/LAPSE:2023.8650
Original Submitter
Auto Uploader for LAPSE
Links to Related Works