LAPSE:2023.7085
Published Article
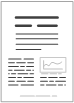
LAPSE:2023.7085
Coal Mine Belt Conveyor Foreign Objects Recognition Method of Improved YOLOv5 Algorithm with Defogging and Deblurring
February 24, 2023
The belt conveyor is the main equipment for underground coal transportation. Its coal flow is mixed with large coal, gangue, anchor rods, wooden strips, and other foreign objects, which easily causes failure of the conveyor belt, such as scratching, tearing, and even broken belts. Aiming at the problem that it was difficult to accurately identify the foreign objects of underground belt conveyors due to the influence of fog, high-speed operation, and obscuration, the coal mine belt conveyor foreign object recognition method of improved YOLOv5 algorithm with defogging and deblurring was proposed. In order to improve the clarity of the monitoring video of the belt conveyor, the dark channel priori defogging algorithm is applied to reduce the impact of fog on the clarity of the monitoring video, and the image is sharpened by user-defined convolution method to reduce the blurring effect on the image in high-speed operation condition. In order to improve the precision of foreign object identification, the convolution block attention module is used to improve the feature expression ability of the foreign object in the complex background. Through adaptive spatial feature fusion, the multi-layer feature information of the foreign object image is more fully fused so as to achieve the goal of accurate recognition of foreign objects. In order to verify the recognition effect of the improved YOLOv5 algorithm, a comparative test is conducted with self-built data set and a public data set. The results show that the performance of the improved YOLOv5 algorithm is better than SSD, YOLOv3, and YOLOv5. The belt conveyor monitoring video of resolution for 1920 × 1080 in Huangling Coal Mine is used for identification verification, the recognition accuracy can reach 95.09%, and the recognition frame rate is 56.50 FPS. The improved YOLOv5 algorithm can provide a reference for the accurate recognition of targets in a complex underground environment.
Record ID
Keywords
adaptive spatial feature fusion, attention mechanism, belt conveyor, deep learning, foreign objects recognition, YOLOv5
Subject
Suggested Citation
Mao Q, Li S, Hu X, Xue X. Coal Mine Belt Conveyor Foreign Objects Recognition Method of Improved YOLOv5 Algorithm with Defogging and Deblurring. (2023). LAPSE:2023.7085
Author Affiliations
Mao Q: School of Mechanical Engineering, Xi’an University of Science and Technology, Xi’an 710054, China; Shaanxi Key Laboratory of Mine Electromechanical Equipment Intelligent Detection and Control, Xi’an 710054, China
Li S: School of Mechanical Engineering, Xi’an University of Science and Technology, Xi’an 710054, China; Shaanxi Key Laboratory of Mine Electromechanical Equipment Intelligent Detection and Control, Xi’an 710054, China [ORCID]
Hu X: School of Mechanical Engineering, Xi’an University of Science and Technology, Xi’an 710054, China; Shaanxi Key Laboratory of Mine Electromechanical Equipment Intelligent Detection and Control, Xi’an 710054, China
Xue X: School of Mechanical Engineering, Xi’an University of Science and Technology, Xi’an 710054, China; Shaanxi Key Laboratory of Mine Electromechanical Equipment Intelligent Detection and Control, Xi’an 710054, China
Li S: School of Mechanical Engineering, Xi’an University of Science and Technology, Xi’an 710054, China; Shaanxi Key Laboratory of Mine Electromechanical Equipment Intelligent Detection and Control, Xi’an 710054, China [ORCID]
Hu X: School of Mechanical Engineering, Xi’an University of Science and Technology, Xi’an 710054, China; Shaanxi Key Laboratory of Mine Electromechanical Equipment Intelligent Detection and Control, Xi’an 710054, China
Xue X: School of Mechanical Engineering, Xi’an University of Science and Technology, Xi’an 710054, China; Shaanxi Key Laboratory of Mine Electromechanical Equipment Intelligent Detection and Control, Xi’an 710054, China
Journal Name
Energies
Volume
15
Issue
24
First Page
9504
Year
2022
Publication Date
2022-12-14
Published Version
ISSN
1996-1073
Version Comments
Original Submission
Other Meta
PII: en15249504, Publication Type: Journal Article
Record Map
Published Article
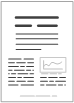
LAPSE:2023.7085
This Record
External Link
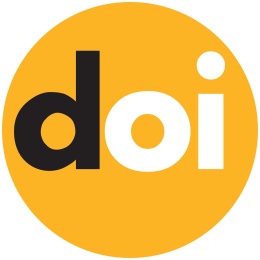
doi:10.3390/en15249504
Publisher Version
Download
Meta
Record Statistics
Record Views
50
Version History
[v1] (Original Submission)
Feb 24, 2023
Verified by curator on
Feb 24, 2023
This Version Number
v1
Citations
Most Recent
This Version
URL Here
https://psecommunity.org/LAPSE:2023.7085
Original Submitter
Auto Uploader for LAPSE
Links to Related Works