LAPSE:2023.6347
Published Article
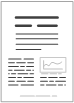
LAPSE:2023.6347
Fault Diagnosis of Wind Turbine Bearings Based on CEEMDAN-GWO-KELM
February 23, 2023
To solve the problem of fault signals of wind turbine bearings being weak, not easy to extract, and difficult to identify, this paper proposes a fault diagnosis method for fan bearings based on Complete Ensemble Empirical Mode Decomposition with Adaptive Noise (CEEMDAN) and Grey Wolf Algorithm Optimization Kernel Extreme Learning Machine (GWO-KELM). First, eliminating the interference of noise on the collected vibration signal should be conducted, in which the wavelet threshold denoising approach is used in order to reduce the noise interference with the vibration signal. Next, CEEMDAN is used to decompose the signal after a denoising operation to obtain the multi-group intrinsic mode function (IMF), and the feature vector is selected by combining the correlation coefficients to eliminate the spurious feature components. Finally, the fuzzy entropy for the chosen IMF component is input into the GWO-KELM model as a feature vector for defect detection. After diagnosing the Case Western Reserve University (CWRU) dataset by the method presented in this research, it is found that the method can identify 99.42% of the various bearing states. When compared to existing combination approaches, the proposed method is shown to be more efficient for diagnosing wind turbine bearing faults.
Record ID
Keywords
bearings, CEEMDAN, fault diagnosis, fuzzy entropy, GWO-KELM, wind turbine
Subject
Suggested Citation
Liu L, Wei Y, Song X, Zhang L. Fault Diagnosis of Wind Turbine Bearings Based on CEEMDAN-GWO-KELM. (2023). LAPSE:2023.6347
Author Affiliations
Liu L: College of Artificial Intelligence, North China University of Science and Technology, Tangshan 063210, China; College of Mechanical and Energy Engineering, Shanghai Technical Institute of Electronics & Information, Shanghai 201411, China
Wei Y: College of Artificial Intelligence, North China University of Science and Technology, Tangshan 063210, China
Song X: Faculty of International Languages, Qinggong College, North China University of Science and Technology, Tangshan 064000, China
Zhang L: College of Metallurgy and Energy, North China University of Science and Technology, Tangshan 063009, China
Wei Y: College of Artificial Intelligence, North China University of Science and Technology, Tangshan 063210, China
Song X: Faculty of International Languages, Qinggong College, North China University of Science and Technology, Tangshan 064000, China
Zhang L: College of Metallurgy and Energy, North China University of Science and Technology, Tangshan 063009, China
Journal Name
Energies
Volume
16
Issue
1
First Page
48
Year
2022
Publication Date
2022-12-21
Published Version
ISSN
1996-1073
Version Comments
Original Submission
Other Meta
PII: en16010048, Publication Type: Journal Article
Record Map
Published Article
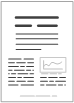
LAPSE:2023.6347
This Record
External Link
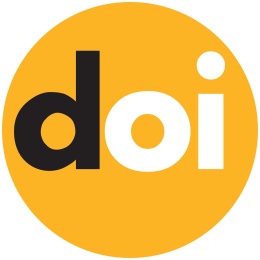
doi:10.3390/en16010048
Publisher Version
Download
Meta
Record Statistics
Record Views
39
Version History
[v1] (Original Submission)
Feb 23, 2023
Verified by curator on
Feb 23, 2023
This Version Number
v1
Citations
Most Recent
This Version
URL Here
https://psecommunity.org/LAPSE:2023.6347
Original Submitter
Auto Uploader for LAPSE
Links to Related Works