LAPSE:2023.6194
Published Article
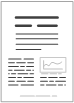
LAPSE:2023.6194
Control of Hybrid Electric Vehicle Powertrain Using Offline-Online Hybrid Reinforcement Learning
February 23, 2023
Hybrid electric vehicles can achieve better fuel economy than conventional vehicles by utilizing multiple power sources. While these power sources have been controlled by rule-based or optimization-based control algorithms, recent studies have shown that machine learning-based control algorithms such as online Deep Reinforcement Learning (DRL) can effectively control the power sources as well. However, the optimization and training processes for the online DRL-based powertrain control strategy can be very time and resource intensive. In this paper, a new offline−online hybrid DRL strategy is presented where offline vehicle data are exploited to build an initial model and an online learning algorithm explores a new control policy to further improve the fuel economy. In this manner, it is expected that the agent can learn an environment consisting of the vehicle dynamics in a given driving condition more quickly compared to the online algorithms, which learn the optimal control policy by interacting with the vehicle model from zero initial knowledge. By incorporating a priori offline knowledge, the simulation results show that the proposed approach not only accelerates the learning process and makes the learning process more stable, but also leads to a better fuel economy compared to online only learning algorithms.
Record ID
Keywords
hybrid electric vehicle, powertrain control, reinforcement learning
Subject
Suggested Citation
Yao Z, Yoon HS, Hong YK. Control of Hybrid Electric Vehicle Powertrain Using Offline-Online Hybrid Reinforcement Learning. (2023). LAPSE:2023.6194
Author Affiliations
Yao Z: Department of Mechanical Engineering, The University of Alabama, Tuscaloosa, AL 35487, USA
Yoon HS: Department of Mechanical Engineering, The University of Alabama, Tuscaloosa, AL 35487, USA [ORCID]
Hong YK: Department of Electrical and Computer Engineering, The University of Alabama, Tuscaloosa, AL 35487, USA
Yoon HS: Department of Mechanical Engineering, The University of Alabama, Tuscaloosa, AL 35487, USA [ORCID]
Hong YK: Department of Electrical and Computer Engineering, The University of Alabama, Tuscaloosa, AL 35487, USA
Journal Name
Energies
Volume
16
Issue
2
First Page
652
Year
2023
Publication Date
2023-01-05
Published Version
ISSN
1996-1073
Version Comments
Original Submission
Other Meta
PII: en16020652, Publication Type: Journal Article
Record Map
Published Article
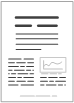
LAPSE:2023.6194
This Record
External Link
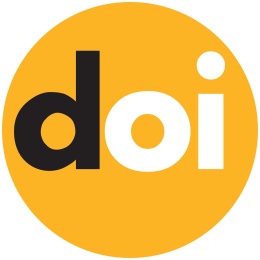
doi:10.3390/en16020652
Publisher Version
Download
Meta
Record Statistics
Record Views
39
Version History
[v1] (Original Submission)
Feb 23, 2023
Verified by curator on
Feb 23, 2023
This Version Number
v1
Citations
Most Recent
This Version
URL Here
https://psecommunity.org/LAPSE:2023.6194
Original Submitter
Auto Uploader for LAPSE
Links to Related Works