LAPSE:2023.5907
Published Article
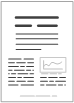
LAPSE:2023.5907
HCRNNIDS: Hybrid Convolutional Recurrent Neural Network-Based Network Intrusion Detection System
February 23, 2023
Nowadays, network attacks are the most crucial problem of modern society. All networks, from small to large, are vulnerable to network threats. An intrusion detection (ID) system is critical for mitigating and identifying malicious threats in networks. Currently, deep learning (DL) and machine learning (ML) are being applied in different domains, especially information security, for developing effective ID systems. These ID systems are capable of detecting malicious threats automatically and on time. However, malicious threats are occurring and changing continuously, so the network requires a very advanced security solution. Thus, creating an effective and smart ID system is a massive research problem. Various ID datasets are publicly available for ID research. Due to the complex nature of malicious attacks with a constantly changing attack detection mechanism, publicly existing ID datasets must be modified systematically on a regular basis. So, in this paper, a convolutional recurrent neural network (CRNN) is used to create a DL-based hybrid ID framework that predicts and classifies malicious cyberattacks in the network. In the HCRNNIDS, the convolutional neural network (CNN) performs convolution to capture local features, and the recurrent neural network (RNN) captures temporal features to improve the ID system’s performance and prediction. To assess the efficacy of the hybrid convolutional recurrent neural network intrusion detection system (HCRNNIDS), experiments were done on publicly available ID data, specifically the modern and realistic CSE-CIC-DS2018 data. The simulation outcomes prove that the proposed HCRNNIDS substantially outperforms current ID methodologies, attaining a high malicious attack detection rate accuracy of up to 97.75% for CSE-CIC-IDS2018 data with 10-fold cross-validation.
Record ID
Keywords
Big Data, convolutional neural network, deep learning, intrusion detection system, Machine Learning, recurrent neural network
Suggested Citation
Khan MA. HCRNNIDS: Hybrid Convolutional Recurrent Neural Network-Based Network Intrusion Detection System. (2023). LAPSE:2023.5907
Author Affiliations
Khan MA: IoT and Big-Data Research Center, Department of Electronics Engineering, Incheon National University, Incheon 2012, Korea [ORCID]
Journal Name
Processes
Volume
9
Issue
5
First Page
834
Year
2021
Publication Date
2021-05-10
Published Version
ISSN
2227-9717
Version Comments
Original Submission
Other Meta
PII: pr9050834, Publication Type: Journal Article
Record Map
Published Article
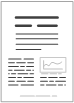
LAPSE:2023.5907
This Record
External Link
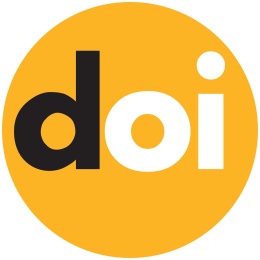
doi:10.3390/pr9050834
Publisher Version
Download
Meta
Record Statistics
Record Views
65
Version History
[v1] (Original Submission)
Feb 23, 2023
Verified by curator on
Feb 23, 2023
This Version Number
v1
Citations
Most Recent
This Version
URL Here
https://psecommunity.org/LAPSE:2023.5907
Original Submitter
Auto Uploader for LAPSE
Links to Related Works