LAPSE:2023.5081
Published Article
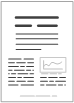
LAPSE:2023.5081
Data-Driven State Prediction and Sensor Fault Diagnosis for Multi-Agent Systems with Application to a Twin Rotational Inverted Pendulum
February 23, 2023
When a multi-agent system is subjected to faults, it is necessary to detect and classify the faults in time. This paper is motivated to propose a data-driven state prediction and sensor fault classification technique. Firstly, neural network-based state prediction model is trained through historical input and output data of the system. Then, the trained model is implemented to the real-time system to predict the system state and output in absence of fault. By comparing the predicted healthy output and the measured output, which can be abnormal in case of sensor faults, a residual signal can be generated. When a sensor fault occurs, the residual signal exceeds the threshold, a fault classification technique is triggered to distinguish fault types. Finally, the designed data-driven state prediction and fault classification algorithms are verified through a twin rotational inverted pendulum system with leader-follower mechanism.
Record ID
Keywords
data-driven, fault classification, multi-agent system, state prediction
Subject
Suggested Citation
Lu X, Liu X, Li B, Zhong J. Data-Driven State Prediction and Sensor Fault Diagnosis for Multi-Agent Systems with Application to a Twin Rotational Inverted Pendulum. (2023). LAPSE:2023.5081
Author Affiliations
Lu X: Sino-German College of Intelligent Manufacturing, Shenzhen Technology University, Shenzhen 518118, China [ORCID]
Liu X: Sino-German College of Intelligent Manufacturing, Shenzhen Technology University, Shenzhen 518118, China [ORCID]
Li B: School of Computer Science, Nanjing University of Posts and Telecommunications, Nanjing 210023, China
Zhong J: College of Mathematics and Computer Science, Zhejiang Normal University, Jinhua 321004, China [ORCID]
Liu X: Sino-German College of Intelligent Manufacturing, Shenzhen Technology University, Shenzhen 518118, China [ORCID]
Li B: School of Computer Science, Nanjing University of Posts and Telecommunications, Nanjing 210023, China
Zhong J: College of Mathematics and Computer Science, Zhejiang Normal University, Jinhua 321004, China [ORCID]
Journal Name
Processes
Volume
9
Issue
9
First Page
1505
Year
2021
Publication Date
2021-08-26
Published Version
ISSN
2227-9717
Version Comments
Original Submission
Other Meta
PII: pr9091505, Publication Type: Journal Article
Record Map
Published Article
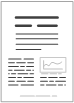
LAPSE:2023.5081
This Record
External Link
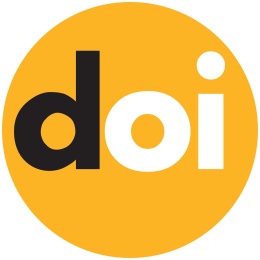
doi:10.3390/pr9091505
Publisher Version
Download
Meta
Record Statistics
Record Views
83
Version History
[v1] (Original Submission)
Feb 23, 2023
Verified by curator on
Feb 23, 2023
This Version Number
v1
Citations
Most Recent
This Version
URL Here
https://psecommunity.org/LAPSE:2023.5081
Original Submitter
Auto Uploader for LAPSE
Links to Related Works