LAPSE:2023.36903v1
Published Article
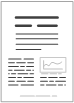
LAPSE:2023.36903v1
A Hybrid Cluster Variational Autoencoder Model for Monitoring the Multimode Blast Furnace System
November 30, 2023
Efficient monitoring of the blast furnace system is crucial for maintaining high production efficiency and ensuring product quality. This article introduces a hybrid cluster variational autoencoder model for monitoring the blast furnace ironmaking process which exhibits multimode behaviors. In contrast to traditional approaches, this method utilizes neural networks to learn data features and effectively handles the diverse feature types observed in different production modes. Through the utilization of a clustering process within the hidden layer of the variational autoencoder, the proposed technique facilitates efficient fault detection in the context of multimodal blast furnace data. Based on the variational autoencoder model, this study further establishes a unified monitoring index and defines a method for computing the control limits. The application of the model to real blast furnace data reveals its proficiency in accurately identifying faults across diverse modes; compared with the probabilistic principal component analysis based on the local nearest neighbor standardization method and the recursive probabilistic principal component analysis, the model shows a reduction in false positives by up to 10.3% and a substantial reduction of 19.2% in the missed detection rate. This method achieves a remarkable false detection rate of only 0.2% and 0 instances of missed detection.
Record ID
Keywords
Gaussian mixture model, multimode blast furnace system, process monitoring, variational autoencoder
Subject
Suggested Citation
Chen C, Cai J. A Hybrid Cluster Variational Autoencoder Model for Monitoring the Multimode Blast Furnace System. (2023). LAPSE:2023.36903v1
Author Affiliations
Chen C: College of Metrology and Measurement Engineering, China Jiliang University, Hangzhou 310018, China
Cai J: College of Metrology and Measurement Engineering, China Jiliang University, Hangzhou 310018, China [ORCID]
Cai J: College of Metrology and Measurement Engineering, China Jiliang University, Hangzhou 310018, China [ORCID]
Journal Name
Processes
Volume
11
Issue
9
First Page
2580
Year
2023
Publication Date
2023-08-29
Published Version
ISSN
2227-9717
Version Comments
Original Submission
Other Meta
PII: pr11092580, Publication Type: Journal Article
Record Map
Published Article
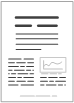
LAPSE:2023.36903v1
This Record
External Link
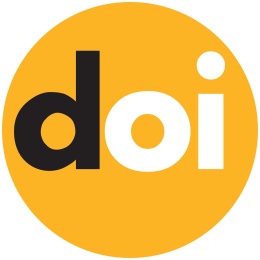
doi:10.3390/pr11092580
Publisher Version
Download
Meta
Record Statistics
Record Views
107
Version History
[v1] (Original Submission)
Nov 30, 2023
Verified by curator on
Nov 30, 2023
This Version Number
v1
Citations
Most Recent
This Version
URL Here
https://psecommunity.org/LAPSE:2023.36903v1
Original Submitter
Calvin Tsay
Links to Related Works