LAPSE:2023.36868
Published Article
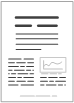
LAPSE:2023.36868
A Novel Hybrid Optimization Approach for Fault Detection in Photovoltaic Arrays and Inverters Using AI and Statistical Learning Techniques: A Focus on Sustainable Environment
November 30, 2023
Fault detection in PV arrays and inverters is critical for ensuring maximum efficiency and performance. Artificial intelligence (AI) learning can be used to quickly identify issues, resulting in a sustainable environment with reduced downtime and maintenance costs. As the use of solar energy systems continues to grow, the need for reliable and efficient fault detection and diagnosis techniques becomes more critical. This paper presents a novel approach for fault detection in photovoltaic (PV) arrays and inverters, combining AI techniques. It integrates Elman neural network (ENN), boosted tree algorithms (BTA), multi-layer perceptron (MLP), and Gaussian processes regression (GPR) for enhanced accuracy and reliability in fault diagnosis. It leverages its strengths for the accuracy and reliability of fault diagnosis. Feature engineering-based sensitivity analysis was utilized for feature extraction. The fault detection and diagnosis were assessed using several statistical criteria including PBAIS, MAE, NSE, RMSE, and MAPE. Two intelligent learning scenarios are carried out. The first scenario is conducted for PV array fault detection with DC power (DCP) as output. The second scenario is conducted for inverter fault detection with AC power (ACP) as the output. The proposed technique is capable of detecting faults in PV arrays and inverters, providing a reliable solution for enhancing the performance and reliability of solar energy systems. A real-world solar energy dataset is used to evaluate the proposed technique with results compared to existing detection techniques and obtained results showing that it outperforms existing fault detection techniques, achieving higher accuracy and better performance. The GPR-M4 optimization justified its reliably among all the models with MAPE = 0.0393 and MAE = 0.002 for inverter fault detection, and MAPE = 0.091 and MAE = 0.000 for PV array fault detection.
Record ID
Keywords
Artificial Intelligence, boosted tree algorithms, Elman neural network, Fault Detection, Gaussian processes regression, multi-layer perceptron, sustainable development
Suggested Citation
Abubakar A, Jibril MM, Almeida CFM, Gemignani M, Yahya MN, Abba SI. A Novel Hybrid Optimization Approach for Fault Detection in Photovoltaic Arrays and Inverters Using AI and Statistical Learning Techniques: A Focus on Sustainable Environment. (2023). LAPSE:2023.36868
Author Affiliations
Abubakar A: Department of Electrical Engineering and Automation, Escola Politecnica da Universidade de São Paulo, São Paulo 05508-010, SP, Brazil [ORCID]
Jibril MM: Department of Civil Engineering, Kano University of Science and Technology (KUST), Wudil 713101, Nigeria [ORCID]
Almeida CFM: Department of Electrical Engineering and Automation, Escola Politecnica da Universidade de São Paulo, São Paulo 05508-010, SP, Brazil
Gemignani M: Department of Electrical Engineering and Automation, Escola Politecnica da Universidade de São Paulo, São Paulo 05508-010, SP, Brazil [ORCID]
Yahya MN: Department of Agricultural and Environmental Engineering, Bayero University, PMB 3011, Gwarzo Road, Kano 700271, Nigeria
Abba SI: Interdisciplinary Research Center for Membrane and Water Security, King Fahd University of Petroleum and Minerals, Dhahran 31261, Saudi Arabia
Jibril MM: Department of Civil Engineering, Kano University of Science and Technology (KUST), Wudil 713101, Nigeria [ORCID]
Almeida CFM: Department of Electrical Engineering and Automation, Escola Politecnica da Universidade de São Paulo, São Paulo 05508-010, SP, Brazil
Gemignani M: Department of Electrical Engineering and Automation, Escola Politecnica da Universidade de São Paulo, São Paulo 05508-010, SP, Brazil [ORCID]
Yahya MN: Department of Agricultural and Environmental Engineering, Bayero University, PMB 3011, Gwarzo Road, Kano 700271, Nigeria
Abba SI: Interdisciplinary Research Center for Membrane and Water Security, King Fahd University of Petroleum and Minerals, Dhahran 31261, Saudi Arabia
Journal Name
Processes
Volume
11
Issue
9
First Page
2549
Year
2023
Publication Date
2023-08-25
Published Version
ISSN
2227-9717
Version Comments
Original Submission
Other Meta
PII: pr11092549, Publication Type: Journal Article
Record Map
Published Article
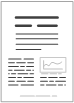
LAPSE:2023.36868
This Record
External Link
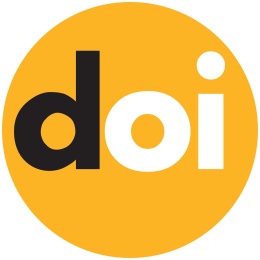
doi:10.3390/pr11092549
Publisher Version
Download
Meta
Record Statistics
Record Views
64
Version History
[v1] (Original Submission)
Nov 30, 2023
Verified by curator on
Nov 30, 2023
This Version Number
v1
Citations
Most Recent
This Version
URL Here
https://psecommunity.org/LAPSE:2023.36868
Original Submitter
Calvin Tsay
Links to Related Works