LAPSE:2023.36807
Published Article
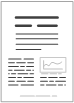
LAPSE:2023.36807
Gout Staging Diagnosis Method Based on Deep Reinforcement Learning
September 21, 2023
In clinical practice, diseases with a prolonged course and disease characteristics at the time of diagnosis are often classified into specific stages. The precision of disease staging significantly impacts the therapeutic and curative outcomes for patients, and the diagnosis of multi-clinical-stage diseases based on electronic medical records is a problem that needs further research. Gout is a multi-stage disease. This paper focuses on the research of gout and proposes a staging diagnosis method for gout based on deep reinforcement learning. This method firstly uses the candidate binary classification model library for accurate diagnosis of gout, and then corrects the results of the binary classification through the set medical rules for diagnosis of gout, and then uses the machine learning model to diagnose different stages of corrected accurate data. In the course of the experiment, deep reinforcement learning was introduced to solve the hyperparameter tuning problem of the staging model. Through experiments conducted on 24,872 electronic medical records, the accuracy rate of gout diagnosis was found to be 90.03%, while the accuracy rate for diagnosing different stages of gout disease reached 86.85%. These findings serve as a valuable tool in assisting clinicians with accurate staging and diagnosis of gout. The application of deep reinforcement learning in gout staging diagnosis demonstrates a significant enhancement in diagnostic accuracy, thereby validating the effectiveness and feasibility of this method.
Record ID
Keywords
deep reinforcement learning, disease staging diagnosis, electronic medical records, gout
Subject
Suggested Citation
Ma C, Pan C, Ye Z, Ren H, Huang H, Qu J. Gout Staging Diagnosis Method Based on Deep Reinforcement Learning. (2023). LAPSE:2023.36807
Author Affiliations
Ma C: School of Computer Science and Technology, Harbin University of Science and Technology, Harbin 150080, China
Pan C: School of Computer Science and Technology, Harbin University of Science and Technology, Harbin 150080, China
Ye Z: School of Computer Science and Technology, Harbin University of Science and Technology, Harbin 150080, China
Ren H: School of Computer Science and Technology, Harbin University of Science and Technology, Harbin 150080, China
Huang H: School of Computer Science and Technology, Harbin University of Science and Technology, Harbin 150080, China
Qu J: Heilongjiang Province Cyberspace Research Center, Harbin 150090, China
Pan C: School of Computer Science and Technology, Harbin University of Science and Technology, Harbin 150080, China
Ye Z: School of Computer Science and Technology, Harbin University of Science and Technology, Harbin 150080, China
Ren H: School of Computer Science and Technology, Harbin University of Science and Technology, Harbin 150080, China
Huang H: School of Computer Science and Technology, Harbin University of Science and Technology, Harbin 150080, China
Qu J: Heilongjiang Province Cyberspace Research Center, Harbin 150090, China
Journal Name
Processes
Volume
11
Issue
8
First Page
2450
Year
2023
Publication Date
2023-08-15
Published Version
ISSN
2227-9717
Version Comments
Original Submission
Other Meta
PII: pr11082450, Publication Type: Journal Article
Record Map
Published Article
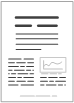
LAPSE:2023.36807
This Record
External Link
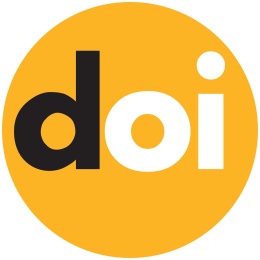
doi:10.3390/pr11082450
Publisher Version
Download
Meta
Record Statistics
Record Views
114
Version History
[v1] (Original Submission)
Sep 21, 2023
Verified by curator on
Sep 21, 2023
This Version Number
v1
Citations
Most Recent
This Version
URL Here
https://psecommunity.org/LAPSE:2023.36807
Original Submitter
Calvin Tsay
Links to Related Works