LAPSE:2023.36797
Published Article
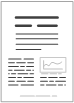
LAPSE:2023.36797
Bearing Fault Diagnosis Based on ICEEMDAN Deep Learning Network
September 21, 2023
Bearing fault diagnosis has evolved from machine learning to deep learning, addressing the issues of performance degradation in deep learning networks and the potential loss of key feature information. This paper proposes a fault diagnosis method for rolling bearing faults based on ICEEMDAN combined with the Hilbert transform (ICEEMDAN-Hilbert) and a residual network (ResNet). Firstly, the collected fault vibration signals are classified as fault samples and randomly sampled with a fixed length. The IMF components obtained by decomposing the bearing fault vibration signals using ICEEMDAN are able to maximize the restoration of fault vibrations. Then, the IMF components are transformed from one-dimensional time-domain signals to two-dimensional time-frequency domain images using Hilbert transformation. The RGB color images can be directly used in deep learning models without the need for manual labeling of a large amount of data, thereby avoiding the loss of key feature information. The ResNet network incorporates the attention mechanism (CBAM) structure for the precise extraction of fault features, enabling a more detailed classification of fault features. Additionally, the residual network effectively addresses the problem of performance degradation in multi-layer network models. Finally, transfer learning is applied in the deep learning network by freezing the training layer parameters and training the fully connected layer. This effectively solves the problem of insufficient data in real operating conditions, which hinders deep training of the model, while also reducing the training time. By combining the ResNet network with the convolutional block attention module (CBAM) structure, the model completes the recognition and training of time-frequency images for rolling bearing faults. The results demonstrate that the ResNet with CBAM model has strong fault feature extraction capabilities, achieving higher accuracy, 7−12% higher than other conventional network models, and exhibiting superior diagnostic performance compared to other deep learning models.
Record ID
Keywords
convolutional block attention module, deep learning, deep residual network, Hilbert, ICEEMDAN, rolling bearing fault diagnosis
Subject
Suggested Citation
Liang B, Feng W. Bearing Fault Diagnosis Based on ICEEMDAN Deep Learning Network. (2023). LAPSE:2023.36797
Author Affiliations
Liang B: School of Marine Engineering Equipment, University of Zhejiang Ocean, Zhoushan 316022, China
Feng W: School of Marine Engineering Equipment, University of Zhejiang Ocean, Zhoushan 316022, China [ORCID]
Feng W: School of Marine Engineering Equipment, University of Zhejiang Ocean, Zhoushan 316022, China [ORCID]
Journal Name
Processes
Volume
11
Issue
8
First Page
2440
Year
2023
Publication Date
2023-08-14
Published Version
ISSN
2227-9717
Version Comments
Original Submission
Other Meta
PII: pr11082440, Publication Type: Journal Article
Record Map
Published Article
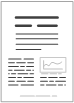
LAPSE:2023.36797
This Record
External Link
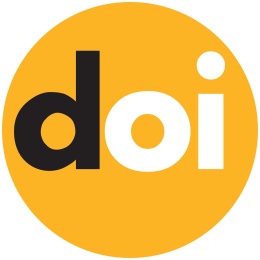
doi:10.3390/pr11082440
Publisher Version
Download
Meta
Record Statistics
Record Views
118
Version History
[v1] (Original Submission)
Sep 21, 2023
Verified by curator on
Sep 21, 2023
This Version Number
v1
Citations
Most Recent
This Version
URL Here
https://psecommunity.org/LAPSE:2023.36797
Original Submitter
Calvin Tsay
Links to Related Works