LAPSE:2023.36771
Published Article
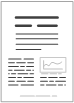
LAPSE:2023.36771
Triaxial Compression Strength Prediction of Fissured Rocks in Deep-Buried Coal Mines Based on an Improved Back Propagation Neural Network Model
September 21, 2023
In deep coal mine strata, characterized by high ground stress and extensive fracturing, predicting the strength of fractured rock masses is crucial for stability analysis of the surrounding rock in coal mine strata. In this study, rock samples were obtained from construction sites in deep coal mine strata and intact, as well as fissured, rock specimens were prepared and subjected to triaxial compression tests. A numerical model based on the discrete element method was then established and the micro-parameters were calibrated. A total of 288 triaxial compression tests on the rock specimens under different conditions of confining pressure, loading rate, fissure dip angle, and fissure length, were conducted to obtain the triaxial compressive strength of the fractured rock specimens under different conditions. To address the limitations of traditional back propagation (BP) neural networks in solving stochastic problems, a modified BP neural network model was developed using a random factor and an interlayer mean square error corrected network model evaluation function. The traditional and modified BP neural network models were then employed to predict the triaxial compressive strength of the fractured rock specimens. Through comparative analysis, it was found that the modified BP neural network prediction model exhibited smaller errors and significantly reduced overfitting, making it an effective tool for predicting the strength of fractured rocks in deep coal mine strata.
Record ID
Keywords
fissured rock specimen, improved BP neural network prediction model, numerical tests, triaxial compression tests
Suggested Citation
Wang Y, Tang B, Tao W, Yuan A, Li T, Liu Z, Zhang F, Mao A. Triaxial Compression Strength Prediction of Fissured Rocks in Deep-Buried Coal Mines Based on an Improved Back Propagation Neural Network Model. (2023). LAPSE:2023.36771
Author Affiliations
Wang Y: State Key Laboratory of Mining Response and Disaster Prevention and Control in Deep Coal Mines, Anhui University of Science and Technology, 168 Taifeng St., Huainan 232001, China; School of Civil Engineering and Architecture, Anhui University of Science a
Tang B: State Key Laboratory of Mining Response and Disaster Prevention and Control in Deep Coal Mines, Anhui University of Science and Technology, 168 Taifeng St., Huainan 232001, China; School of Civil Engineering and Architecture, Anhui University of Science a [ORCID]
Tao W: Anhui Transport Consulting and Design Institute, Hefei 230088, China
Yuan A: School of Civil Engineering and Architecture, Anhui University of Science and Technology, 168 Taifeng St., Huainan 232001, China
Li T: State Key Laboratory of Mining Response and Disaster Prevention and Control in Deep Coal Mines, Anhui University of Science and Technology, 168 Taifeng St., Huainan 232001, China
Liu Z: State Key Laboratory of Mining Response and Disaster Prevention and Control in Deep Coal Mines, Anhui University of Science and Technology, 168 Taifeng St., Huainan 232001, China
Zhang F: State Key Laboratory of Mining Response and Disaster Prevention and Control in Deep Coal Mines, Anhui University of Science and Technology, 168 Taifeng St., Huainan 232001, China
Mao A: State Key Laboratory of Mining Response and Disaster Prevention and Control in Deep Coal Mines, Anhui University of Science and Technology, 168 Taifeng St., Huainan 232001, China
Tang B: State Key Laboratory of Mining Response and Disaster Prevention and Control in Deep Coal Mines, Anhui University of Science and Technology, 168 Taifeng St., Huainan 232001, China; School of Civil Engineering and Architecture, Anhui University of Science a [ORCID]
Tao W: Anhui Transport Consulting and Design Institute, Hefei 230088, China
Yuan A: School of Civil Engineering and Architecture, Anhui University of Science and Technology, 168 Taifeng St., Huainan 232001, China
Li T: State Key Laboratory of Mining Response and Disaster Prevention and Control in Deep Coal Mines, Anhui University of Science and Technology, 168 Taifeng St., Huainan 232001, China
Liu Z: State Key Laboratory of Mining Response and Disaster Prevention and Control in Deep Coal Mines, Anhui University of Science and Technology, 168 Taifeng St., Huainan 232001, China
Zhang F: State Key Laboratory of Mining Response and Disaster Prevention and Control in Deep Coal Mines, Anhui University of Science and Technology, 168 Taifeng St., Huainan 232001, China
Mao A: State Key Laboratory of Mining Response and Disaster Prevention and Control in Deep Coal Mines, Anhui University of Science and Technology, 168 Taifeng St., Huainan 232001, China
Journal Name
Processes
Volume
11
Issue
8
First Page
2414
Year
2023
Publication Date
2023-08-10
Published Version
ISSN
2227-9717
Version Comments
Original Submission
Other Meta
PII: pr11082414, Publication Type: Journal Article
Record Map
Published Article
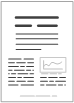
LAPSE:2023.36771
This Record
External Link
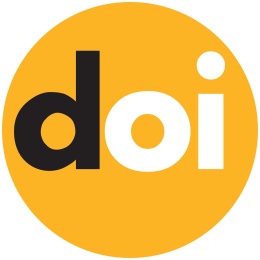
doi:10.3390/pr11082414
Publisher Version
Download
Meta
Record Statistics
Record Views
93
Version History
[v1] (Original Submission)
Sep 21, 2023
Verified by curator on
Sep 21, 2023
This Version Number
v1
Citations
Most Recent
This Version
URL Here
https://psecommunity.org/LAPSE:2023.36771
Original Submitter
Calvin Tsay
Links to Related Works