LAPSE:2023.36744
Published Article
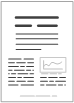
LAPSE:2023.36744
Intelligent Optimization Design of Distillation Columns Using Surrogate Models Based on GA-BP
September 21, 2023
The design of distillation columns significantly impacts the economy, energy consumption, and environment of chemical processes. However, optimizing the design of distillation columns is a very challenging problem. In order to develop an intelligent technique to obtain the best design solution, improve design efficiency, and minimize reliance on experience in the design process, a design methodology based on the GA-BP model is proposed in this paper. Firstly, a distillation column surrogate model is established using the back propagation neural network technique based on the training data from the rigorous simulation, which covers all possible changes in feed conditions, operating conditions, and design parameters. The essence of this step is to turn the distillation design process from model-driven to data-driven. Secondly, the model takes the minimum TAC as the objective function and performs the optimization search using a Genetic Algorithm to obtain the design solution with the minimum TAC, in which a life-cycle assessment (LCA) model is incorporated to evaluate the obtained optimized design solution from both economic and environmental aspects. Finally, the feasibility of the proposed method is verified with a propylene distillation column as an example. The results show that the method has advantages in convergence speed without sacrificing accuracy and can obtain an improved design solution with reduced cost and environmental impact. Compared with the original design using rigorous simulation, the TAC is reduced by 6.1% and carbon emission by 27.13 kgCO2/t.
Record ID
Keywords
BP neural network, distillation column, Genetic Algorithm, intelligent design, life cycle assessment, surrogate modeling
Subject
Suggested Citation
Ye L, Zhang N, Li G, Gu D, Lu J, Lou Y. Intelligent Optimization Design of Distillation Columns Using Surrogate Models Based on GA-BP. (2023). LAPSE:2023.36744
Author Affiliations
Ye L: Innovation Centre for Environment and Resources, School of Chemistry and Chemical Engineering, Shanghai University of Engineering Science, No.333 Longteng Road, Songjiang District, Shanghai 201620, China
Zhang N: Centre for Process Integration, Department of Chemical Engineering and Analytical Science, The University of Manchester, Manchester M13 9PL, UK [ORCID]
Li G: Innovation Centre for Environment and Resources, School of Chemistry and Chemical Engineering, Shanghai University of Engineering Science, No.333 Longteng Road, Songjiang District, Shanghai 201620, China
Gu D: Innovation Centre for Environment and Resources, School of Chemistry and Chemical Engineering, Shanghai University of Engineering Science, No.333 Longteng Road, Songjiang District, Shanghai 201620, China
Lu J: Innovation Centre for Environment and Resources, School of Chemistry and Chemical Engineering, Shanghai University of Engineering Science, No.333 Longteng Road, Songjiang District, Shanghai 201620, China [ORCID]
Lou Y: Innovation Centre for Environment and Resources, School of Chemistry and Chemical Engineering, Shanghai University of Engineering Science, No.333 Longteng Road, Songjiang District, Shanghai 201620, China
Zhang N: Centre for Process Integration, Department of Chemical Engineering and Analytical Science, The University of Manchester, Manchester M13 9PL, UK [ORCID]
Li G: Innovation Centre for Environment and Resources, School of Chemistry and Chemical Engineering, Shanghai University of Engineering Science, No.333 Longteng Road, Songjiang District, Shanghai 201620, China
Gu D: Innovation Centre for Environment and Resources, School of Chemistry and Chemical Engineering, Shanghai University of Engineering Science, No.333 Longteng Road, Songjiang District, Shanghai 201620, China
Lu J: Innovation Centre for Environment and Resources, School of Chemistry and Chemical Engineering, Shanghai University of Engineering Science, No.333 Longteng Road, Songjiang District, Shanghai 201620, China [ORCID]
Lou Y: Innovation Centre for Environment and Resources, School of Chemistry and Chemical Engineering, Shanghai University of Engineering Science, No.333 Longteng Road, Songjiang District, Shanghai 201620, China
Journal Name
Processes
Volume
11
Issue
8
First Page
2386
Year
2023
Publication Date
2023-08-08
Published Version
ISSN
2227-9717
Version Comments
Original Submission
Other Meta
PII: pr11082386, Publication Type: Journal Article
Record Map
Published Article
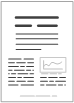
LAPSE:2023.36744
This Record
External Link
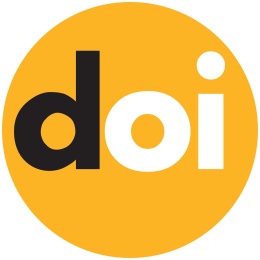
doi:10.3390/pr11082386
Publisher Version
Download
Meta
Record Statistics
Record Views
114
Version History
[v1] (Original Submission)
Sep 21, 2023
Verified by curator on
Sep 21, 2023
This Version Number
v1
Citations
Most Recent
This Version
URL Here
https://psecommunity.org/LAPSE:2023.36744
Original Submitter
Calvin Tsay
Links to Related Works