LAPSE:2023.36652
Published Article
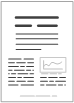
LAPSE:2023.36652
An Interpretable Predictive Model for Health Aspects of Solvents via Rough Set Theory
September 20, 2023
This paper presents a machine learning (ML) approach to predict the potential health issues of solvents by uncovering the hidden relationship between substances and toxicity. Solvent selection is a crucial step in industrial processes. However, prolonged exposure to solvents has been found to pose significant risks to human health. To mitigate these hazards, it is crucial to develop a predictive model for health performance by identifying the contributing factors to solvent toxicity. This research aims to develop a predictive model for health issues related to solvent toxicity. Among various algorithms in ML, Rough Set Machine Learning (RSML) was chosen for this work due to its interpretable nature of the generated models. The models have been developed through data collection on the toxicity of various organic solvents, the construction of predictive models with decision rules, and model verification. The results reveal correlations between solvent toxicity and the Balaban index, valence connectivity index, Wiener index, and boiling points. The generated predictive model using RSML has successfully provided insightful observations about the correlation between human toxicity and molecular attributes.
Record ID
Keywords
health indices, Machine Learning, organic solvents, rough set theory, rough set-based machine learning
Subject
Suggested Citation
Hoo WY, Ooi J, Chemmangattuvalappil NG, Chong JW, Lim CH, Eden MR. An Interpretable Predictive Model for Health Aspects of Solvents via Rough Set Theory. (2023). LAPSE:2023.36652
Author Affiliations
Hoo WY: School of Engineering and Physical Sciences, Heriot-Watt University Malaysia, No. 1, Jalan Venna P5/2, Precinct 5, Putrajaya 62200, Malaysia
Ooi J: School of Engineering and Physical Sciences, Heriot-Watt University Malaysia, No. 1, Jalan Venna P5/2, Precinct 5, Putrajaya 62200, Malaysia
Chemmangattuvalappil NG: Department of Chemical & Environmental Engineering, University of Nottingham Malaysia, Jalan Broga, Semenyih 43500, Malaysia [ORCID]
Chong JW: Department of Chemical & Environmental Engineering, University of Nottingham Malaysia, Jalan Broga, Semenyih 43500, Malaysia
Lim CH: School of Engineering and Physical Sciences, Heriot-Watt University Malaysia, No. 1, Jalan Venna P5/2, Precinct 5, Putrajaya 62200, Malaysia [ORCID]
Eden MR: Department of Chemical Engineering, Auburn University, Auburn, AL 36849, USA [ORCID]
Ooi J: School of Engineering and Physical Sciences, Heriot-Watt University Malaysia, No. 1, Jalan Venna P5/2, Precinct 5, Putrajaya 62200, Malaysia
Chemmangattuvalappil NG: Department of Chemical & Environmental Engineering, University of Nottingham Malaysia, Jalan Broga, Semenyih 43500, Malaysia [ORCID]
Chong JW: Department of Chemical & Environmental Engineering, University of Nottingham Malaysia, Jalan Broga, Semenyih 43500, Malaysia
Lim CH: School of Engineering and Physical Sciences, Heriot-Watt University Malaysia, No. 1, Jalan Venna P5/2, Precinct 5, Putrajaya 62200, Malaysia [ORCID]
Eden MR: Department of Chemical Engineering, Auburn University, Auburn, AL 36849, USA [ORCID]
Journal Name
Processes
Volume
11
Issue
8
First Page
2293
Year
2023
Publication Date
2023-07-31
Published Version
ISSN
2227-9717
Version Comments
Original Submission
Other Meta
PII: pr11082293, Publication Type: Journal Article
Record Map
Published Article
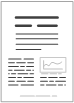
LAPSE:2023.36652
This Record
External Link
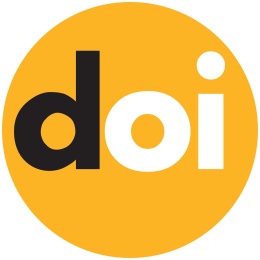
doi:10.3390/pr11082293
Publisher Version
Download
Meta
Record Statistics
Record Views
100
Version History
[v1] (Original Submission)
Sep 20, 2023
Verified by curator on
Sep 20, 2023
This Version Number
v1
Citations
Most Recent
This Version
URL Here
https://psecommunity.org/LAPSE:2023.36652
Original Submitter
Calvin Tsay
Links to Related Works