LAPSE:2023.36632
Published Article
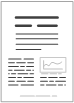
LAPSE:2023.36632
Failure Risk Prediction Model for Girth Welds in High-Strength Steel Pipeline Based on Historical Data and Artificial Neural Network
September 20, 2023
Pipelines are the most economical and sensible way to transport oil and gas. Long-distance oil and gas pipelines consist of many steel pipes or pipe fittings joined by welded girth welds, so girth welds are an essential part of pipelines. Owing to the limitations of welding conditions and the complexity of controlling weld quality in the field, some defects are inevitably present in girth welds and adjacent weld areas. These defects can lead to pipeline safety problems; therefore, it is necessary to perform failure risk assessment of pipeline girth welds. In this study, an artificial neural network model was proposed to predict the failure risk of pipeline girth welds with defects. Firstly, many pipeline girth weld failure cases, pipeline excavation, and inspection data were collected and analyzed to determine the main factors influencing girth weld failure. Secondly, a spatial orthogonal optimization method was used to select training samples for the artificial neural network model to ensure that the training sample set could cover the feature space with a minimum number of samples. Thirdly, a prediction model based on BP neural networks was established to predict the failure risk levels. The training dataset/testing dataset was 602/4215, and the prediction accuracy for all risks of girth welds achieved an acceptable level. This study can provide a valuable reference for pipeline operators to prevent pipeline accidents.
Record ID
Keywords
failure risk, girth welds, pipeline, sample selection
Suggested Citation
Wang K, Zhang M, Guo Q, Ma W, Zhang Y, Wu W. Failure Risk Prediction Model for Girth Welds in High-Strength Steel Pipeline Based on Historical Data and Artificial Neural Network. (2023). LAPSE:2023.36632
Author Affiliations
Wang K: School of Materials Science and Engineering, Xi’an University of Technology, Xi’an 710048, China; Tubular Goods Research Institute of CNPC, Xi’an 710077, China
Zhang M: School of Materials Science and Engineering, Xi’an University of Technology, Xi’an 710048, China [ORCID]
Guo Q: Shaanxi City Gas Industry Development Co., Ltd., Xi’an 710048, China
Ma W: Tubular Goods Research Institute of CNPC, Xi’an 710077, China
Zhang Y: School of Chemical Engineering, Northwest University, Xi’an 710069, China [ORCID]
Wu W: School of Chemical Engineering, Northwest University, Xi’an 710069, China [ORCID]
Zhang M: School of Materials Science and Engineering, Xi’an University of Technology, Xi’an 710048, China [ORCID]
Guo Q: Shaanxi City Gas Industry Development Co., Ltd., Xi’an 710048, China
Ma W: Tubular Goods Research Institute of CNPC, Xi’an 710077, China
Zhang Y: School of Chemical Engineering, Northwest University, Xi’an 710069, China [ORCID]
Wu W: School of Chemical Engineering, Northwest University, Xi’an 710069, China [ORCID]
Journal Name
Processes
Volume
11
Issue
8
First Page
2273
Year
2023
Publication Date
2023-07-28
Published Version
ISSN
2227-9717
Version Comments
Original Submission
Other Meta
PII: pr11082273, Publication Type: Journal Article
Record Map
Published Article
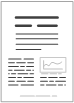
LAPSE:2023.36632
This Record
External Link
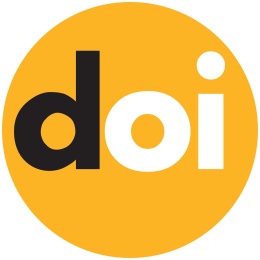
doi:10.3390/pr11082273
Publisher Version
Download
Meta
Record Statistics
Record Views
87
Version History
[v1] (Original Submission)
Sep 20, 2023
Verified by curator on
Sep 20, 2023
This Version Number
v1
Citations
Most Recent
This Version
URL Here
https://psecommunity.org/LAPSE:2023.36632
Original Submitter
Calvin Tsay
Links to Related Works