LAPSE:2023.36585
Published Article
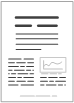
LAPSE:2023.36585
EW-YOLOv7: A Lightweight and Effective Detection Model for Small Defects in Electrowetting Display
September 20, 2023
In order to overcome the shortcomings of existing electrowetting display defect detection models in terms of computational complexity, structural complexity, detection speed, and detection accuracy, this article proposes an improved YOLOv7-based electrowetting display defect detection model. The model effectively optimizes the detection performance of display defects, especially small target defects, by integrating GhostNetV2 modules, Acmix attention mechanisms, and NGWD (Normalized Gaussian Wasserstein Distance) Loss. At the same time, it reduces the parameter size of the network model and improves the inference efficiency of the network. This article evaluates the performance of an improved model using a self-constructed electrowetting display defect dataset. The experimental results show that the proposed improved model achieves an average detection rate (mAP) of 89.5% and an average inference time of 35.9 ms. Compared to the original network, the number of parameters and computational costs are reduced by 19.2% and 64.3%, respectively. Compared with current state-of-the-art detection network models, the proposed EW-YOLOv7 exhibits superior performance in detecting electrowetting display defects. This model helps to solve the problem of defect detection in industrial production of electrowetting display and assists the research team in quickly identifying the causes and locations of defects.
Record ID
Keywords
detection model, electrowetting display, GhostNetV2, small defects, YOLOv7
Subject
Suggested Citation
Zheng Z, Chen N, Wu J, Xv Z, Liu S, Luo Z. EW-YOLOv7: A Lightweight and Effective Detection Model for Small Defects in Electrowetting Display. (2023). LAPSE:2023.36585
Author Affiliations
Zheng Z: College of Information Science and Technology, Zhongkai University of Agriculture and Engineering, Guangzhou 510225, China
Chen N: College of Information Science and Technology, Zhongkai University of Agriculture and Engineering, Guangzhou 510225, China
Wu J: College of Information Science and Technology, Zhongkai University of Agriculture and Engineering, Guangzhou 510225, China
Xv Z: College of Information Science and Technology, Zhongkai University of Agriculture and Engineering, Guangzhou 510225, China
Liu S: College of Information Science and Technology, Zhongkai University of Agriculture and Engineering, Guangzhou 510225, China; Intelligent Agriculture Engineering Research Center, Zhongkai University of Agriculture and Engineering, Guangzhou 510225, China; G
Luo Z: College of Information Science and Technology, Zhongkai University of Agriculture and Engineering, Guangzhou 510225, China; Intelligent Agriculture Engineering Research Center, Zhongkai University of Agriculture and Engineering, Guangzhou 510225, China; G
Chen N: College of Information Science and Technology, Zhongkai University of Agriculture and Engineering, Guangzhou 510225, China
Wu J: College of Information Science and Technology, Zhongkai University of Agriculture and Engineering, Guangzhou 510225, China
Xv Z: College of Information Science and Technology, Zhongkai University of Agriculture and Engineering, Guangzhou 510225, China
Liu S: College of Information Science and Technology, Zhongkai University of Agriculture and Engineering, Guangzhou 510225, China; Intelligent Agriculture Engineering Research Center, Zhongkai University of Agriculture and Engineering, Guangzhou 510225, China; G
Luo Z: College of Information Science and Technology, Zhongkai University of Agriculture and Engineering, Guangzhou 510225, China; Intelligent Agriculture Engineering Research Center, Zhongkai University of Agriculture and Engineering, Guangzhou 510225, China; G
Journal Name
Processes
Volume
11
Issue
7
First Page
2037
Year
2023
Publication Date
2023-07-07
Published Version
ISSN
2227-9717
Version Comments
Original Submission
Other Meta
PII: pr11072037, Publication Type: Journal Article
Record Map
Published Article
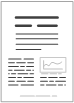
LAPSE:2023.36585
This Record
External Link
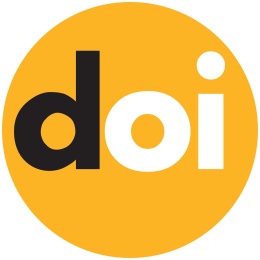
doi:10.3390/pr11072037
Publisher Version
Download
Meta
Record Statistics
Record Views
92
Version History
[v1] (Original Submission)
Sep 20, 2023
Verified by curator on
Sep 20, 2023
This Version Number
v1
Citations
Most Recent
This Version
URL Here
https://psecommunity.org/LAPSE:2023.36585
Original Submitter
Calvin Tsay
Links to Related Works