LAPSE:2023.36563
Published Article
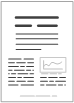
LAPSE:2023.36563
Efficient Multi-Objective Optimization on Dynamic Flexible Job Shop Scheduling Using Deep Reinforcement Learning Approach
August 3, 2023
Previous research focuses on approaches of deep reinforcement learning (DRL) to optimize diverse types of the single-objective dynamic flexible job shop scheduling problem (DFJSP), e.g., energy consumption, earliness and tardiness penalty and machine utilization rate, which gain many improvements in terms of objective metrics in comparison with metaheuristic algorithms such as GA (genetic algorithm) and dispatching rules such as MRT (most remaining time first). However, single-objective optimization in the job shop floor cannot satisfy the requirements of modern smart manufacturing systems, and the multiple-objective DFJSP has become mainstream and the core of intelligent workshops. A complex production environment in a real-world factory causes scheduling entities to have sophisticated characteristics, e.g., a job’s non-uniform processing time, uncertainty of the operation number and restraint of the due time, avoidance of the single machine’s prolonged slack time as well as overweight load, which make a method of the combination of dispatching rules in DRL brought up to adapt to the manufacturing environment at different rescheduling points and accumulate maximum rewards for a global optimum. In our work, we apply the structure of a dual layer DDQN (DLDDQN) to solve the DFJSP in real time with new job arrivals, and two objectives are optimized simultaneously, i.e., the minimization of the delay time sum and makespan. The framework includes two layers (agents): the higher one is named as a goal selector, which utilizes DDQN as a function approximator for selecting one reward form from six proposed ones that embody the two optimization objectives, while the lower one, called an actuator, utilizes DDQN to decide on an optimal rule that has a maximum Q value. The generated benchmark instances trained in our framework converged perfectly, and the comparative experiments validated the superiority and generality of the proposed DLDDQN.
Record ID
Keywords
deep reinforcement learning, delay time sum, dual layer deep Q-network, dynamic flexible job shop scheduling, global optimum, makespan, multi-objective optimization
Subject
Suggested Citation
Wu Z, Fan H, Sun Y, Peng M. Efficient Multi-Objective Optimization on Dynamic Flexible Job Shop Scheduling Using Deep Reinforcement Learning Approach. (2023). LAPSE:2023.36563
Author Affiliations
Wu Z: Faculty of Information Engineering and Automation, Kunming University of Science and Technology, Kunming 650504, China [ORCID]
Fan H: Faculty of Information Engineering and Automation, Kunming University of Science and Technology, Kunming 650504, China; Faculty of Modern Agricultural Engineering, Kunming University of Science and Technology, Kunming 650500, China
Sun Y: Faculty of Information Engineering and Automation, Kunming University of Science and Technology, Kunming 650504, China
Peng M: Faculty of Information Engineering and Automation, Kunming University of Science and Technology, Kunming 650504, China
Fan H: Faculty of Information Engineering and Automation, Kunming University of Science and Technology, Kunming 650504, China; Faculty of Modern Agricultural Engineering, Kunming University of Science and Technology, Kunming 650500, China
Sun Y: Faculty of Information Engineering and Automation, Kunming University of Science and Technology, Kunming 650504, China
Peng M: Faculty of Information Engineering and Automation, Kunming University of Science and Technology, Kunming 650504, China
Journal Name
Processes
Volume
11
Issue
7
First Page
2018
Year
2023
Publication Date
2023-07-06
Published Version
ISSN
2227-9717
Version Comments
Original Submission
Other Meta
PII: pr11072018, Publication Type: Journal Article
Record Map
Published Article
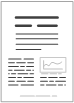
LAPSE:2023.36563
This Record
External Link
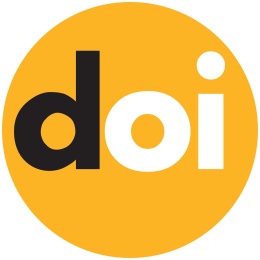
doi:10.3390/pr11072018
Publisher Version
Download
Meta
Record Statistics
Record Views
126
Version History
[v1] (Original Submission)
Aug 3, 2023
Verified by curator on
Aug 3, 2023
This Version Number
v1
Citations
Most Recent
This Version
URL Here
https://psecommunity.org/LAPSE:2023.36563
Original Submitter
Calvin Tsay
Links to Related Works