LAPSE:2023.36477
Published Article
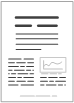
LAPSE:2023.36477
Gearbox Fault Diagnosis Based on Optimized Stacked Denoising Auto Encoder and Kernel Extreme Learning Machine
August 2, 2023
The gearbox is one of the key components of many large mechanical transmission devices. Due to the complex working environment, the vibration signal stability of the gear box is poor, the fault feature extraction is difficult, and the fault diagnosis accuracy makes it difficult to meet the expected requirements. To solve this problem, this paper proposes a gearbox fault diagnosis method based on an optimized stacked denoising auto encoder (SDAE) and kernel extreme learning machine (KELM). Firstly, the particle swarm optimization algorithm in adaptive weight (SAPSO) was adopted to optimize the SDAE network structure, and the number of hidden layer nodes, learning rate, noise addition ratio and iteration times were adaptively obtained to make SDAE obtain the best network structure. Then, the best SDAE network structure was used to extract the deep feature information of weak faults in the original signal. Finally, the extracted fault features are fed into KELM for fault classification. Experimental results show that the classification accuracy of the proposed method can reach 97.2% under the condition of low signal-to-noise ratio, which shows the effectiveness and robustness of the proposed method compared with other diagnostic methods.
Record ID
Keywords
fault diagnosis, gearbox, kernel extreme learning machine, stacked denoising automatic encoder
Subject
Suggested Citation
Wu Z, Yan H, Zhan X, Wen L, Jia X. Gearbox Fault Diagnosis Based on Optimized Stacked Denoising Auto Encoder and Kernel Extreme Learning Machine. (2023). LAPSE:2023.36477
Author Affiliations
Wu Z: Shijiazhuang Campus, Army Engineering University of PLA, Shijiazhuang 050003, China
Yan H: Shijiazhuang Campus, Army Engineering University of PLA, Shijiazhuang 050003, China
Zhan X: Shijiazhuang Campus, Army Engineering University of PLA, Shijiazhuang 050003, China; Hebei Key Laboratory of Condition Monitoring and Assessment of Mechanical Equipment, Shijiazhuang 050003, China
Wen L: Shijiazhuang Campus, Army Engineering University of PLA, Shijiazhuang 050003, China
Jia X: Shijiazhuang Campus, Army Engineering University of PLA, Shijiazhuang 050003, China
Yan H: Shijiazhuang Campus, Army Engineering University of PLA, Shijiazhuang 050003, China
Zhan X: Shijiazhuang Campus, Army Engineering University of PLA, Shijiazhuang 050003, China; Hebei Key Laboratory of Condition Monitoring and Assessment of Mechanical Equipment, Shijiazhuang 050003, China
Wen L: Shijiazhuang Campus, Army Engineering University of PLA, Shijiazhuang 050003, China
Jia X: Shijiazhuang Campus, Army Engineering University of PLA, Shijiazhuang 050003, China
Journal Name
Processes
Volume
11
Issue
7
First Page
1936
Year
2023
Publication Date
2023-06-27
Published Version
ISSN
2227-9717
Version Comments
Original Submission
Other Meta
PII: pr11071936, Publication Type: Journal Article
Record Map
Published Article
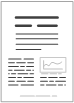
LAPSE:2023.36477
This Record
External Link
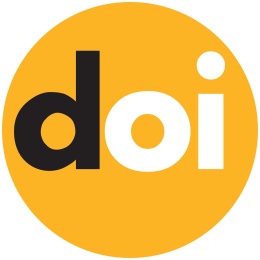
doi:10.3390/pr11071936
Publisher Version
Download
Meta
Record Statistics
Record Views
74
Version History
[v1] (Original Submission)
Aug 2, 2023
Verified by curator on
Aug 2, 2023
This Version Number
v1
Citations
Most Recent
This Version
URL Here
https://psecommunity.org/LAPSE:2023.36477
Original Submitter
Calvin Tsay
Links to Related Works