LAPSE:2023.36470
Published Article
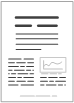
LAPSE:2023.36470
Fault Diagnosis of Rotating Machinery Bearings Based on Improved DCNN and WOA-DELM
August 2, 2023
A bearing is a critical component in the transmission of rotating machinery. However, due to prolonged exposure to heavy loads and high-speed environments, rolling bearings are highly susceptible to faults, Hence, it is crucial to enhance bearing fault diagnosis to ensure safe and reliable operation of rotating machinery. In order to achieve this, a rotating machinery fault diagnosis method based on a deep convolutional neural network (DCNN) and Whale Optimization Algorithm (WOA) optimized Deep Extreme Learning Machine (DELM) is proposed in this paper. DCNN is a combination of the Efficient Channel Attention Net (ECA-Net) and Bi-directional Long Short-Term Memory (BiLSTM). In this method, firstly, a DCNN classification network is constructed. The ECA-Net and BiLSTM are brought into the deep convolutional neural network to extract critical features. Next, the WOA is used to optimize the weight of the initial input layer of DELM to build the WOA-DELM classifier model. Finally, the features extracted by the Improved DCNN (IDCNN) are sent to the WOA-DELM model for bearing fault diagnosis. The diagnostic capability of the proposed IDCNN-WOA-DELM method was evaluated through multiple-condition fault diagnosis experiments using the CWRU-bearing dataset with various settings, and comparative tests against other methods were conducted as well. The results indicate that the proposed method demonstrates good diagnostic performance.
Record ID
Keywords
Bi-directional Long Short-Term Memory, convolutional neural network, DELM, Efficient Channel Attention Module, fault diagnosis, rotating machinery
Subject
Suggested Citation
Wang L, Ping D, Wang C, Jiang S, Shen J, Zhang J. Fault Diagnosis of Rotating Machinery Bearings Based on Improved DCNN and WOA-DELM. (2023). LAPSE:2023.36470
Author Affiliations
Wang L: School of Mechanical Engineering, North China University of Water Resources and Electric Power, Zhengzhou 450045, China
Ping D: School of Mechanical Engineering, North China University of Water Resources and Electric Power, Zhengzhou 450045, China
Wang C: School of Management and Economics, North China University of Water Resources and Electric Power, Zhengzhou 450045, China [ORCID]
Jiang S: School of Mechanical Engineering, North China University of Water Resources and Electric Power, Zhengzhou 450045, China
Shen J: School of Mechanical Engineering, North China University of Water Resources and Electric Power, Zhengzhou 450045, China
Zhang J: School of Computing, Engineering & Digital Technologies, Teesside University, Middlesbrough TS1 3BA, UK [ORCID]
Ping D: School of Mechanical Engineering, North China University of Water Resources and Electric Power, Zhengzhou 450045, China
Wang C: School of Management and Economics, North China University of Water Resources and Electric Power, Zhengzhou 450045, China [ORCID]
Jiang S: School of Mechanical Engineering, North China University of Water Resources and Electric Power, Zhengzhou 450045, China
Shen J: School of Mechanical Engineering, North China University of Water Resources and Electric Power, Zhengzhou 450045, China
Zhang J: School of Computing, Engineering & Digital Technologies, Teesside University, Middlesbrough TS1 3BA, UK [ORCID]
Journal Name
Processes
Volume
11
Issue
7
First Page
1928
Year
2023
Publication Date
2023-06-26
Published Version
ISSN
2227-9717
Version Comments
Original Submission
Other Meta
PII: pr11071928, Publication Type: Journal Article
Record Map
Published Article
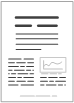
LAPSE:2023.36470
This Record
External Link
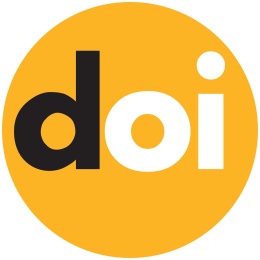
doi:10.3390/pr11071928
Publisher Version
Download
Meta
Record Statistics
Record Views
88
Version History
[v1] (Original Submission)
Aug 2, 2023
Verified by curator on
Aug 2, 2023
This Version Number
v1
Citations
Most Recent
This Version
URL Here
https://psecommunity.org/LAPSE:2023.36470
Original Submitter
Calvin Tsay
Links to Related Works