LAPSE:2023.36459
Published Article
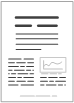
LAPSE:2023.36459
Droplet Based Estimation of Viscosity of Water−PVP Solutions Using Convolutional Neural Networks
August 2, 2023
The viscosity of a liquid is the property that measures the liquid’s internal resistance to flow. Monitoring viscosity is a vital component of quality control in several industrial fields, including chemical, pharmaceutical, food, and energy-related industries. In many industries, the most commonly used instrument for measuring viscosity is capillary viscometers, but their cost and complexity pose challenges for these industries where accurate and real-time viscosity information is vital. In this work, we prepared fourteen solutions with different water and PVP (Polyvinylpyrrolidone) ratios, measured their different viscosity values, and produced videos of their droplets. We extracted the images of the fully developed droplets from the videos and we used the images to train a convolutional neural network model to estimate the viscosity values of the water−PVP solutions. The proposed model was able to accurately estimate the viscosity values of samples of unseen chemical formulations with the same composition with a low MSE score of 0.0243 and R2 score of 0.9576. The proposed method has potential applications in scenarios where real-time monitoring of liquid viscosity is required.
Record ID
Keywords
convolutional neural networks, Polyvinylpyrrolidone, viscosity, viscosity estimation, water–PVP
Suggested Citation
Mrad MA, Csorba K, Galata DL, Nagy ZK, Charaf H. Droplet Based Estimation of Viscosity of Water−PVP Solutions Using Convolutional Neural Networks. (2023). LAPSE:2023.36459
Author Affiliations
Mrad MA: Department of Automation and Applied Informatics, Faculty of Electrical Engineering and Informatics, Budapest University of Technology and Economics, Műegyetem rkp.3., H-1111 Budapest, Hungary [ORCID]
Csorba K: Department of Automation and Applied Informatics, Faculty of Electrical Engineering and Informatics, Budapest University of Technology and Economics, Műegyetem rkp.3., H-1111 Budapest, Hungary [ORCID]
Galata DL: Department of Organic Chemistry and Technology, Faculty of Chemical Technology and Biotechnology, Budapest University of Technology and Economics, Budafoki út 8., F. II., H-1111 Budapest, Hungary
Nagy ZK: Department of Organic Chemistry and Technology, Faculty of Chemical Technology and Biotechnology, Budapest University of Technology and Economics, Budafoki út 8., F. II., H-1111 Budapest, Hungary [ORCID]
Charaf H: Department of Automation and Applied Informatics, Faculty of Electrical Engineering and Informatics, Budapest University of Technology and Economics, Műegyetem rkp.3., H-1111 Budapest, Hungary
Csorba K: Department of Automation and Applied Informatics, Faculty of Electrical Engineering and Informatics, Budapest University of Technology and Economics, Műegyetem rkp.3., H-1111 Budapest, Hungary [ORCID]
Galata DL: Department of Organic Chemistry and Technology, Faculty of Chemical Technology and Biotechnology, Budapest University of Technology and Economics, Budafoki út 8., F. II., H-1111 Budapest, Hungary
Nagy ZK: Department of Organic Chemistry and Technology, Faculty of Chemical Technology and Biotechnology, Budapest University of Technology and Economics, Budafoki út 8., F. II., H-1111 Budapest, Hungary [ORCID]
Charaf H: Department of Automation and Applied Informatics, Faculty of Electrical Engineering and Informatics, Budapest University of Technology and Economics, Műegyetem rkp.3., H-1111 Budapest, Hungary
Journal Name
Processes
Volume
11
Issue
7
First Page
1917
Year
2023
Publication Date
2023-06-26
Published Version
ISSN
2227-9717
Version Comments
Original Submission
Other Meta
PII: pr11071917, Publication Type: Journal Article
Record Map
Published Article
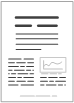
LAPSE:2023.36459
This Record
External Link
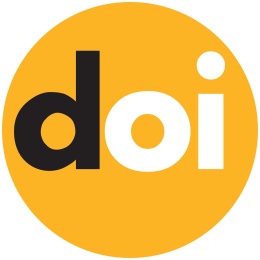
doi:10.3390/pr11071917
Publisher Version
Download
Meta
Record Statistics
Record Views
99
Version History
[v1] (Original Submission)
Aug 2, 2023
Verified by curator on
Aug 2, 2023
This Version Number
v1
Citations
Most Recent
This Version
URL Here
https://psecommunity.org/LAPSE:2023.36459
Original Submitter
Calvin Tsay
Links to Related Works