LAPSE:2023.36373
Published Article
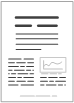
LAPSE:2023.36373
Accelerating SARS-CoV-2 Vaccine Development: Leveraging Novel Hybrid Deep Learning Models and Bioinformatics Analysis for Epitope Selection and Classification
July 13, 2023
It is essential to use highly antigenic epitope areas, since the development of peptide vaccines heavily relies on the precise design of epitope regions that can elicit a strong immune response. Choosing epitope regions experimentally for the production of the SARS-CoV-2 vaccine can be time-consuming, costly, and labor-intensive. Scientists have created in silico prediction techniques based on machine learning to find these regions, to cut down the number of candidate epitopes that might be tested in experiments, and, as a result, to lessen the time-consuming process of their mapping. However, the tools and approaches involved continue to have low accuracy. In this work, we propose a hybrid deep learning model based on a convolutional neural network (CNN) and long short-term memory (LSTM) for the classification of peptides into epitopes or non-epitopes. Numerous transfer learning strategies were utilized, and the fine-tuned method gave the best result, with an AUC of 0.979, an f1 score of 0.902, and 95.1% accuracy, which was far better than the performance of the model trained from scratch. The experimental results obtained show that this model has superior performance when compared to other methods trained on IEDB datasets. Using bioinformatics tools such as ToxinPred, VaxiJen, and AllerTop2.0, the toxicities, antigenicities, and allergenicities, respectively, of the predicted epitopes were determined. In silico cloning and codon optimization were used to successfully express the vaccine in E. coli. This work will help scientists choose the best epitope for the development of the COVID-19 vaccine, reducing cost and labor and thereby accelerating vaccine production.
Record ID
Keywords
COVID-19, deep learning, epitope, transformers, vaccine
Subject
Suggested Citation
Ameen ZS, Mostafa H, Ozsahin DU, Mubarak AS. Accelerating SARS-CoV-2 Vaccine Development: Leveraging Novel Hybrid Deep Learning Models and Bioinformatics Analysis for Epitope Selection and Classification. (2023). LAPSE:2023.36373
Author Affiliations
Ameen ZS: Operational Research Centre in Healthcare, Near East University, TRNC Mersin 10, Nicosia 99138, Turkey
Mostafa H: Department of Information Technology, College of Computer and Information Sciences, Princess Nourah bint Abdulrahman University, P.O. Box 84428, Riyadh 11671, Saudi Arabia
Ozsahin DU: Department of Medical Diagnostic Imaging, College of Health Science, University of Sharjah, Sharjah P.O. Box 27272, United Arab Emirates; Research Institute for Medical and Health Sciences, University of Sharjah, Sharjah P.O. Box 27272, United Arab Emirat
Mubarak AS: Operational Research Centre in Healthcare, Near East University, TRNC Mersin 10, Nicosia 99138, Turkey
Mostafa H: Department of Information Technology, College of Computer and Information Sciences, Princess Nourah bint Abdulrahman University, P.O. Box 84428, Riyadh 11671, Saudi Arabia
Ozsahin DU: Department of Medical Diagnostic Imaging, College of Health Science, University of Sharjah, Sharjah P.O. Box 27272, United Arab Emirates; Research Institute for Medical and Health Sciences, University of Sharjah, Sharjah P.O. Box 27272, United Arab Emirat
Mubarak AS: Operational Research Centre in Healthcare, Near East University, TRNC Mersin 10, Nicosia 99138, Turkey
Journal Name
Processes
Volume
11
Issue
6
First Page
1829
Year
2023
Publication Date
2023-06-16
Published Version
ISSN
2227-9717
Version Comments
Original Submission
Other Meta
PII: pr11061829, Publication Type: Journal Article
Record Map
Published Article
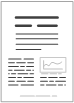
LAPSE:2023.36373
This Record
External Link
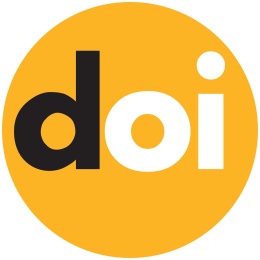
doi:10.3390/pr11061829
Publisher Version
Download
Meta
Record Statistics
Record Views
98
Version History
[v1] (Original Submission)
Jul 13, 2023
Verified by curator on
Jul 13, 2023
This Version Number
v1
Citations
Most Recent
This Version
URL Here
https://psecommunity.org/LAPSE:2023.36373
Original Submitter
Calvin Tsay
Links to Related Works