LAPSE:2023.36314
Published Article
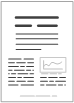
LAPSE:2023.36314
An Artificial Intelligence Method for Flowback Control of Hydraulic Fracturing Fluid in Oil and Gas Wells
July 7, 2023
Hydraulic fracturing is one of the main ways to increase oil and gas production. However, with existing methods, the diameter of the nozzle cannot be easily adjusted. This therefore results in ‘sand production’ in flowback fluid, affecting the application of hydraulic fracturing. This is because it is difficult to identify the one-dimensional series signal of fracturing fluid collected on site. In order to avoid ‘sand production’ in the flowback fluid, the nozzle should be properly controlled. Aiming to address this problem, a novel augmented residual deep learning neural network (AU-RES) is proposed that can identify the characteristics of multiple one-dimensional time series signals and effectively predict the diameter of the nozzle. The AU-RES network includes three parts: signal conversion layer, residual and convolutional layer, fully connected layer (including regression layer). Firstly, a spatial conversion algorithm for multiple one-dimensional time series signals is proposed, which can transform the one-dimensional time series signals into images in high dimensional space. Secondly, the features of the images are extracted and identified by the residual network. Thirdly, the network hyperparameters are optimized to improve the prediction accuracy of the network. Simulations and experiments performed on the field data samples show that the RMSE and LOSS when training the AU-RES network are 0.131 and 0.00021, respectively, and the prediction error of the test samples is 0.1689. In the gas field experiments, fracturing fluid sand production could be controlled, thus demonstrating the validity and reliability of the AU-RES network. By using the AU-RES neural network, sand particles will not be present in the flowback of fracturing fluid, thus improving the efficiency of hydraulic fracturing and reducing the cost of hydraulic fracturing. In addition, the AU-RES network can also be used in other similar situations.
Record ID
Keywords
Artificial Intelligence, deep learning neural network, hydraulic fracture, process control
Suggested Citation
Li R, Wei H, Wang J, Li B, Zheng X, Bai W. An Artificial Intelligence Method for Flowback Control of Hydraulic Fracturing Fluid in Oil and Gas Wells. (2023). LAPSE:2023.36314
Author Affiliations
Li R: Mechanical Engineering College, Xian ShiYou University, Xi’an 710065, China [ORCID]
Wei H: Mechanical Engineering College, Xian ShiYou University, Xi’an 710065, China
Wang J: Mechanical Engineering College, Xian ShiYou University, Xi’an 710065, China
Li B: Mechanical Engineering College, Xian ShiYou University, Xi’an 710065, China
Zheng X: Mechanical Engineering College, Xian ShiYou University, Xi’an 710065, China
Bai W: Mechanical Engineering College, Xian ShiYou University, Xi’an 710065, China
Wei H: Mechanical Engineering College, Xian ShiYou University, Xi’an 710065, China
Wang J: Mechanical Engineering College, Xian ShiYou University, Xi’an 710065, China
Li B: Mechanical Engineering College, Xian ShiYou University, Xi’an 710065, China
Zheng X: Mechanical Engineering College, Xian ShiYou University, Xi’an 710065, China
Bai W: Mechanical Engineering College, Xian ShiYou University, Xi’an 710065, China
Journal Name
Processes
Volume
11
Issue
6
First Page
1773
Year
2023
Publication Date
2023-06-10
Published Version
ISSN
2227-9717
Version Comments
Original Submission
Other Meta
PII: pr11061773, Publication Type: Journal Article
Record Map
Published Article
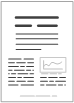
LAPSE:2023.36314
This Record
External Link
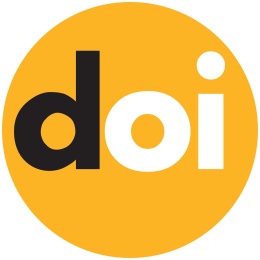
doi:10.3390/pr11061773
Publisher Version
Download
Meta
Record Statistics
Record Views
121
Version History
[v1] (Original Submission)
Jul 7, 2023
Verified by curator on
Jul 7, 2023
This Version Number
v1
Citations
Most Recent
This Version
URL Here
https://psecommunity.org/LAPSE:2023.36314
Original Submitter
Calvin Tsay
Links to Related Works