LAPSE:2023.36077
Published Article
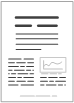
LAPSE:2023.36077
Composite Fault Diagnosis of Aviation Generator Based on EnFWA-DBN
June 9, 2023
Because of the existence of composite faults, which consist of both short-out and eccentricity faults, the characteristics of the output voltage and internal magnetic field of aviation generators are less different than those of single short-out faults; this causes the eccentricity fault to be difficult to identify. In order to solve this problem, this paper proposes a fault diagnosis method using an enhanced fireworks algorithm (EnFWA) to optimize a deep belief network (DBN). The aviation generator model is built according to the finite element method (FEM), whereas the output of different combinations of composite faults are obtained using simulations. The EnFWA algorithm is used to train and optimize the DBN network to obtain the best structure. Meanwhile, an extreme learning machine (ELM) classifier performs fault diagnosis and classification on the test data. The diagnosis results show that a pinpoint accuracy can be achieved using the proposed method in the diagnosis of composite faults in aviation generators.
Record ID
Keywords
composite fault, deep belief network, enhanced fireworks algorithm, extreme learning machine
Subject
Suggested Citation
Yang Z, Bao X, Zhou Q, Yang J. Composite Fault Diagnosis of Aviation Generator Based on EnFWA-DBN. (2023). LAPSE:2023.36077
Author Affiliations
Yang Z: College of Electronic Information and Automation, Civil Aviation University of China, Tianjin 300300, China [ORCID]
Bao X: College of Electronic Information and Automation, Civil Aviation University of China, Tianjin 300300, China
Zhou Q: College of Electronic Information and Automation, Civil Aviation University of China, Tianjin 300300, China
Yang J: Engineering Techniques Training Center, Civil Aviation University of China, Tianjin 300300, China [ORCID]
Bao X: College of Electronic Information and Automation, Civil Aviation University of China, Tianjin 300300, China
Zhou Q: College of Electronic Information and Automation, Civil Aviation University of China, Tianjin 300300, China
Yang J: Engineering Techniques Training Center, Civil Aviation University of China, Tianjin 300300, China [ORCID]
Journal Name
Processes
Volume
11
Issue
5
First Page
1577
Year
2023
Publication Date
2023-05-22
Published Version
ISSN
2227-9717
Version Comments
Original Submission
Other Meta
PII: pr11051577, Publication Type: Journal Article
Record Map
Published Article
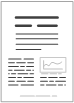
LAPSE:2023.36077
This Record
External Link
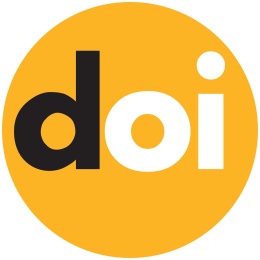
doi:10.3390/pr11051577
Publisher Version
Download
Meta
Record Statistics
Record Views
88
Version History
[v1] (Original Submission)
Jun 9, 2023
Verified by curator on
Jun 9, 2023
This Version Number
v1
Citations
Most Recent
This Version
URL Here
https://psecommunity.org/LAPSE:2023.36077
Original Submitter
Calvin Tsay
Links to Related Works