LAPSE:2023.35952
Published Article
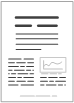
LAPSE:2023.35952
Investigating the Power of LSTM-Based Models in Solar Energy Forecasting
June 7, 2023
Solar is a significant renewable energy source. Solar energy can provide for the world’s energy needs while minimizing global warming from traditional sources. Forecasting the output of renewable energy has a considerable impact on decisions about the operation and management of power systems. It is crucial to accurately forecast the output of renewable energy sources in order to assure grid dependability and sustainability and to reduce the risk and expense of energy markets and systems. Recent advancements in long short-term memory (LSTM) have attracted researchers to the model, and its promising potential is reflected in the method’s richness and the growing number of papers about it. To facilitate further research and development in this area, this paper investigates LSTM models for forecasting solar energy by using time-series data. The paper is divided into two parts: (1) independent LSTM models and (2) hybrid models that incorporate LSTM as another type of technique. The Root mean square error (RMSE) and other error metrics are used as the representative evaluation metrics for comparing the accuracy of the selected methods. According to empirical studies, the two types of models (independent LSTM and hybrid) have distinct advantages and disadvantages depending on the scenario. For instance, LSTM outperforms the other standalone models, but hybrid models generally outperform standalone models despite their longer data training time requirement. The most notable discovery is the better suitability of LSTM as a predictive model to forecast the amount of solar radiation and photovoltaic power compared with other conventional machine learning methods.
Record ID
Keywords
deep learning, hybrid model, long short-term memory, photovoltaic power forecasting, Renewable and Sustainable Energy, solar irradiance forecasting
Subject
Suggested Citation
Jailani NLM, Dhanasegaran JK, Alkawsi G, Alkahtani AA, Phing CC, Baashar Y, Capretz LF, Al-Shetwi AQ, Tiong SK. Investigating the Power of LSTM-Based Models in Solar Energy Forecasting. (2023). LAPSE:2023.35952
Author Affiliations
Jailani NLM: Institute of Sustainable Energy, Universiti Tenaga Nasional, Kajang 43000, Malaysia [ORCID]
Dhanasegaran JK: Institute of Sustainable Energy, Universiti Tenaga Nasional, Kajang 43000, Malaysia
Alkawsi G: Institute of Sustainable Energy, Universiti Tenaga Nasional, Kajang 43000, Malaysia [ORCID]
Alkahtani AA: Institute of Sustainable Energy, Universiti Tenaga Nasional, Kajang 43000, Malaysia [ORCID]
Phing CC: Institute of Sustainable Energy, Universiti Tenaga Nasional, Kajang 43000, Malaysia
Baashar Y: Faculty of Computing and Informatics, Universiti Malaysia Sabah (UMS), Labuan 87000, Malaysia [ORCID]
Capretz LF: Department of Electrical and Computer Engineering, Western University, London, ON N6A 5B9, Canada [ORCID]
Al-Shetwi AQ: Electrical Engineering Department, Fahad Bin Sultan University, Tabuk 71454, Saudi Arabia [ORCID]
Tiong SK: Institute of Sustainable Energy, Universiti Tenaga Nasional, Kajang 43000, Malaysia
Dhanasegaran JK: Institute of Sustainable Energy, Universiti Tenaga Nasional, Kajang 43000, Malaysia
Alkawsi G: Institute of Sustainable Energy, Universiti Tenaga Nasional, Kajang 43000, Malaysia [ORCID]
Alkahtani AA: Institute of Sustainable Energy, Universiti Tenaga Nasional, Kajang 43000, Malaysia [ORCID]
Phing CC: Institute of Sustainable Energy, Universiti Tenaga Nasional, Kajang 43000, Malaysia
Baashar Y: Faculty of Computing and Informatics, Universiti Malaysia Sabah (UMS), Labuan 87000, Malaysia [ORCID]
Capretz LF: Department of Electrical and Computer Engineering, Western University, London, ON N6A 5B9, Canada [ORCID]
Al-Shetwi AQ: Electrical Engineering Department, Fahad Bin Sultan University, Tabuk 71454, Saudi Arabia [ORCID]
Tiong SK: Institute of Sustainable Energy, Universiti Tenaga Nasional, Kajang 43000, Malaysia
Journal Name
Processes
Volume
11
Issue
5
First Page
1382
Year
2023
Publication Date
2023-05-03
Published Version
ISSN
2227-9717
Version Comments
Original Submission
Other Meta
PII: pr11051382, Publication Type: Review
Record Map
Published Article
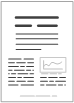
LAPSE:2023.35952
This Record
External Link
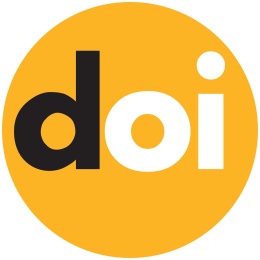
doi:10.3390/pr11051382
Publisher Version
Download
Meta
Record Statistics
Record Views
132
Version History
[v1] (Original Submission)
Jun 7, 2023
Verified by curator on
Jun 7, 2023
This Version Number
v1
Citations
Most Recent
This Version
URL Here
https://psecommunity.org/LAPSE:2023.35952
Original Submitter
Calvin Tsay
Links to Related Works