LAPSE:2023.35759
Published Article
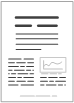
LAPSE:2023.35759
A Parametric Physics-Informed Deep Learning Method for Probabilistic Design of Thermal Protection Systems
May 23, 2023
Precise and efficient calculations are necessary to accurately assess the effects of thermal protection system (TPS) uncertainties on aerospacecrafts. This paper presents a probabilistic design methodology for TPSs based on physics-informed neural networks (PINNs) with parametric uncertainty. A typical thermal coating system is used to investigate the impact of uncertainty on the thermal properties of insulation materials and to evaluate the resulting temperature distribution. A sensitivity analysis is conducted to identify the influence of the parameters on the thermal response. The results show that PINNs can produce quick and accurate predictions of the temperature of insulation materials. The accuracy of the PINN model is comparable to that of a response surface surrogate model. Still, the computational time required by the PINN model is only a fraction of the latter. Considering both computational efficiency and accuracy, the PINN model can be used as a high-precision surrogate model to guide the TPS design effectively.
Record ID
Keywords
physics-informed neural networks, Surrogate Model, thermal protection system, uncertainty quantification
Suggested Citation
Zhang R, Xu N, Zhang K, Wang L, Lu G. A Parametric Physics-Informed Deep Learning Method for Probabilistic Design of Thermal Protection Systems. (2023). LAPSE:2023.35759
Author Affiliations
Zhang R: School of Mathematics and Science, North China Electric Power University, Beijing 102206, China
Xu N: School of Mathematics and Science, North China Electric Power University, Beijing 102206, China
Zhang K: China Academy of Launch Vehicle Technology, Beijing 100076, China
Wang L: School of Mathematics and Science, North China Electric Power University, Beijing 102206, China
Lu G: School of Energy Power and Mechanical Engineering, North China Electric Power University, Beijing 102206, China
Xu N: School of Mathematics and Science, North China Electric Power University, Beijing 102206, China
Zhang K: China Academy of Launch Vehicle Technology, Beijing 100076, China
Wang L: School of Mathematics and Science, North China Electric Power University, Beijing 102206, China
Lu G: School of Energy Power and Mechanical Engineering, North China Electric Power University, Beijing 102206, China
Journal Name
Energies
Volume
16
Issue
9
First Page
3820
Year
2023
Publication Date
2023-04-29
Published Version
ISSN
1996-1073
Version Comments
Original Submission
Other Meta
PII: en16093820, Publication Type: Journal Article
Record Map
Published Article
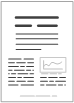
LAPSE:2023.35759
This Record
External Link
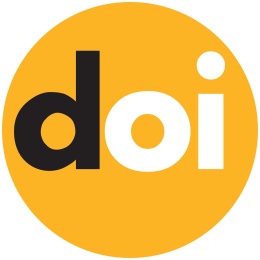
doi:10.3390/en16093820
Publisher Version
Download
Meta
Record Statistics
Record Views
107
Version History
[v1] (Original Submission)
May 23, 2023
Verified by curator on
May 23, 2023
This Version Number
v1
Citations
Most Recent
This Version
URL Here
https://psecommunity.org/LAPSE:2023.35759
Original Submitter
Calvin Tsay
Links to Related Works