LAPSE:2023.35651
Published Article
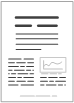
LAPSE:2023.35651
Transformer Winding Fault Classification and Condition Assessment Based on Random Forest Using FRA
May 23, 2023
At present, the condition assessment of transformer winding based on frequency response analysis (FRA) measurements demands skilled personnel. Despite many research efforts in the last decade, there is still no definitive methodology for the interpretation and condition assessment of transformer winding based on FRA results, and this is a major challenge for the industrial application of the FRA method. To overcome this challenge, this paper proposes a transformer condition assessment (TCA) algorithm, which is based on numerical indices, and a supervised machine learning technique to develop a method for the automatic interpretation of FRA results. For this purpose, random forest (RF) classifiers were developed for the first time to identify the condition of transformer winding and classify different faults in the transformer windings. Mainly, six common states of the transformer were classified in this research, i.e., healthy transformer, healthy transformer with saturated core, mechanically damaged winding, short-circuited winding, open-circuited winding, and repeatability issues. In this research, the data from 139 FRA measurements performed in more than 80 power transformers were used. The database belongs to the transformers having different ratings, sizes, designs, and manufacturers. The results reveal that the proposed TCA algorithm can effectively assess the transformer winding condition with up to 93% accuracy without much human intervention.
Record ID
Keywords
condition assessment, decision tree (DT), frequency response analysis (FRA), Machine Learning, numerical indices, power transformer, random forest (RF)
Subject
Suggested Citation
Tahir M, Tenbohlen S. Transformer Winding Fault Classification and Condition Assessment Based on Random Forest Using FRA. (2023). LAPSE:2023.35651
Author Affiliations
Journal Name
Energies
Volume
16
Issue
9
First Page
3714
Year
2023
Publication Date
2023-04-26
Published Version
ISSN
1996-1073
Version Comments
Original Submission
Other Meta
PII: en16093714, Publication Type: Journal Article
Record Map
Published Article
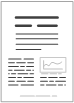
LAPSE:2023.35651
This Record
External Link
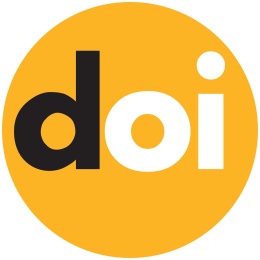
doi:10.3390/en16093714
Publisher Version
Download
Meta
Record Statistics
Record Views
117
Version History
[v1] (Original Submission)
May 23, 2023
Verified by curator on
May 23, 2023
This Version Number
v1
Citations
Most Recent
This Version
URL Here
https://psecommunity.org/LAPSE:2023.35651
Original Submitter
Calvin Tsay
Links to Related Works