LAPSE:2023.35581
Published Article
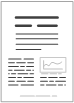
LAPSE:2023.35581
Supervisory Control and Data Acquisition for Fault Diagnosis of Wind Turbines via Deep Transfer Learning
May 23, 2023
The installed wind power capacity is growing worldwide. Remote condition monitoring of wind turbines is employed to achieve higher up-times and lower maintenance costs. Machine learning approaches can be used for detecting developing faults in wind turbines in their earlier occurrence. However, training fault detection models may require large amounts of past and present data. These data are often not available or not representative of the current operation behaviour. These data can be acquired with supervisory control and data acquisition systems. Note also that newly commissioned wind farms lack data from previous operation, whilst older installations may also lack representative working condition data as a result of control software updates or component replacements. After such events, a turbine’s operation behaviour can change significantly so its data are no longer representative of its current behaviour. Therefore, this paper shows that cross−turbine transfer learning can improve the accuracy of fault detection models in turbines with scarce data from supervisory control and data acquisition systems. In particular, it highlights that combining the knowledge from turbines with scarce data and turbines with plentiful data enables earlier detection of faults than prior art methods. In this way, the reuse and the knowledge transfer across wind turbines allows us to overcome this lack of data, thus enabling accurate fault detection in wind turbines.
Record ID
Keywords
condition monitoring, convolutional neural networks, diagnostics, Fault Detection, supervisory control and data acquisition, transfer learning, wind turbines
Subject
Suggested Citation
Simani S, Farsoni S, Castaldi P. Supervisory Control and Data Acquisition for Fault Diagnosis of Wind Turbines via Deep Transfer Learning. (2023). LAPSE:2023.35581
Author Affiliations
Simani S: Department of Engineering, University of Ferrara, 44122 Ferrara, Italy [ORCID]
Farsoni S: Department of Engineering, University of Ferrara, 44122 Ferrara, Italy [ORCID]
Castaldi P: Department of Electrical, Electronic, and Information Engineering, University of Bologna, 40136 Bologna, Italy [ORCID]
Farsoni S: Department of Engineering, University of Ferrara, 44122 Ferrara, Italy [ORCID]
Castaldi P: Department of Electrical, Electronic, and Information Engineering, University of Bologna, 40136 Bologna, Italy [ORCID]
Journal Name
Energies
Volume
16
Issue
9
First Page
3644
Year
2023
Publication Date
2023-04-24
Published Version
ISSN
1996-1073
Version Comments
Original Submission
Other Meta
PII: en16093644, Publication Type: Journal Article
Record Map
Published Article
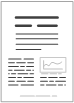
LAPSE:2023.35581
This Record
External Link
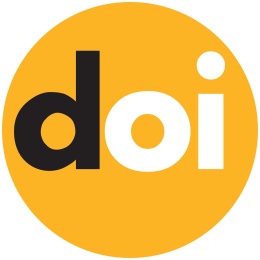
doi:10.3390/en16093644
Publisher Version
Download
Meta
Record Statistics
Record Views
115
Version History
[v1] (Original Submission)
May 23, 2023
Verified by curator on
May 23, 2023
This Version Number
v1
Citations
Most Recent
This Version
URL Here
https://psecommunity.org/LAPSE:2023.35581
Original Submitter
Calvin Tsay
Links to Related Works