LAPSE:2023.35366
Published Article
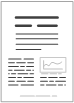
LAPSE:2023.35366
Computational Models That Use a Quantitative Structure−Activity Relationship Approach Based on Deep Learning
April 28, 2023
In the toxicological testing of new small-molecule compounds, it is desirable to establish in silico test methods to predict toxicity instead of relying on animal testing. Since quantitative structure−activity relationships (QSARs) can predict the biological activity from structural information for small-molecule compounds, QSAR applications for in silico toxicity prediction have been studied for a long time. However, in recent years, the remarkable predictive performance of deep learning has attracted attention for practical applications. In this review, we summarize the application of deep learning to QSAR for constructing prediction models, including a discussion of parameter optimization for deep learning.
Record ID
Keywords
bioinformatics, computational models, convolution neural network, deep learning, graph convolutional networks, parameter optimization, quantitative structure–activity relationship
Suggested Citation
Matsuzaka Y, Uesawa Y. Computational Models That Use a Quantitative Structure−Activity Relationship Approach Based on Deep Learning. (2023). LAPSE:2023.35366
Author Affiliations
Matsuzaka Y: Department of Medical Molecular Informatics, Meiji Pharmaceutical University, Kiyose 204-8588, Japan; Division of Molecular and Medical Genetics, Center for Gene and Cell Therapy, The Institute of Medical Science, The University of Tokyo, Minato-ku 108-86
Uesawa Y: Department of Medical Molecular Informatics, Meiji Pharmaceutical University, Kiyose 204-8588, Japan [ORCID]
Uesawa Y: Department of Medical Molecular Informatics, Meiji Pharmaceutical University, Kiyose 204-8588, Japan [ORCID]
Journal Name
Processes
Volume
11
Issue
4
First Page
1296
Year
2023
Publication Date
2023-04-21
Published Version
ISSN
2227-9717
Version Comments
Original Submission
Other Meta
PII: pr11041296, Publication Type: Review
Record Map
Published Article
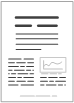
LAPSE:2023.35366
This Record
External Link
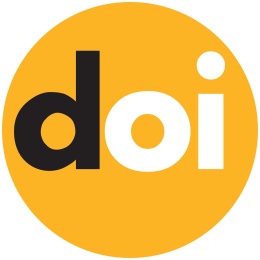
doi:10.3390/pr11041296
Publisher Version
Download
Meta
Record Statistics
Record Views
104
Version History
[v1] (Original Submission)
Apr 28, 2023
Verified by curator on
Apr 28, 2023
This Version Number
v1
Citations
Most Recent
This Version
URL Here
https://psecommunity.org/LAPSE:2023.35366
Original Submitter
Auto Uploader for LAPSE
Links to Related Works