LAPSE:2023.35341
Published Article
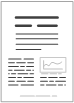
LAPSE:2023.35341
Robustness Prediction in Dynamic Production Processes—A New Surrogate Measure Based on Regression Machine Learning
April 28, 2023
This feasibility study utilized regression models to predict makespan robustness in dynamic production processes with uncertain processing times. Previous methods for robustness determination were computationally intensive (Monte Carlo experiments) or inaccurate (surrogate measures). However, calculating robustness efficiently is crucial for field-synchronous scheduling techniques. Regression models with multiple input features considering uncertain processing times on the critical path outperform traditional surrogate measures. Well-trained regression models internalize the behavior of a dynamic simulation and can quickly predict accurate robustness (correlation: r>0.98). The proposed method was successfully applied to a permutation flow shop scheduling problem, balancing makespan and robustness. Integrating regression models into a metaheuristic model, schedules could be generated that have a similar quality to using Monte Carlo experiments. These results suggest that employing machine learning techniques for robustness prediction could be a promising and efficient alternative to traditional approaches. This work is an addition to our previous extensive study about creating robust stable schedules based on deep reinforcement learning and is part of the applied research project, Predictive Scheduling.
Record ID
Keywords
computational cost reduction, regression model, robust scheduling, stochastic processing times, surrogate measure, uncertainty
Subject
Suggested Citation
Grumbach F, Müller A, Reusch P, Trojahn S. Robustness Prediction in Dynamic Production Processes—A New Surrogate Measure Based on Regression Machine Learning. (2023). LAPSE:2023.35341
Author Affiliations
Grumbach F: Center for Applied Data Science (CfADS), Bielefeld University of Applied Sciences, 33330 Gütersloh, Germany [ORCID]
Müller A: Center for Applied Data Science (CfADS), Bielefeld University of Applied Sciences, 33330 Gütersloh, Germany [ORCID]
Reusch P: Center for Applied Data Science (CfADS), Bielefeld University of Applied Sciences, 33330 Gütersloh, Germany [ORCID]
Trojahn S: Department of Economics, Anhalt University of Applied Sciences, 06406 Bernburg, Germany [ORCID]
Müller A: Center for Applied Data Science (CfADS), Bielefeld University of Applied Sciences, 33330 Gütersloh, Germany [ORCID]
Reusch P: Center for Applied Data Science (CfADS), Bielefeld University of Applied Sciences, 33330 Gütersloh, Germany [ORCID]
Trojahn S: Department of Economics, Anhalt University of Applied Sciences, 06406 Bernburg, Germany [ORCID]
Journal Name
Processes
Volume
11
Issue
4
First Page
1267
Year
2023
Publication Date
2023-04-19
Published Version
ISSN
2227-9717
Version Comments
Original Submission
Other Meta
PII: pr11041267, Publication Type: Journal Article
Record Map
Published Article
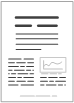
LAPSE:2023.35341
This Record
External Link
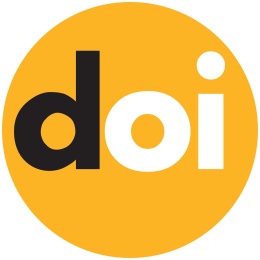
doi:10.3390/pr11041267
Publisher Version
Download
Meta
Record Statistics
Record Views
98
Version History
[v1] (Original Submission)
Apr 28, 2023
Verified by curator on
Apr 28, 2023
This Version Number
v1
Citations
Most Recent
This Version
URL Here
https://psecommunity.org/LAPSE:2023.35341
Original Submitter
Auto Uploader for LAPSE
Links to Related Works