LAPSE:2023.35283
Published Article
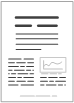
LAPSE:2023.35283
Enhancing Heart Disease Prediction Accuracy through Machine Learning Techniques and Optimization
April 28, 2023
In the medical domain, early identification of cardiovascular issues poses a significant challenge. This study enhances heart disease prediction accuracy using machine learning techniques. Six algorithms (random forest, K-nearest neighbor, logistic regression, Naïve Bayes, gradient boosting, and AdaBoost classifier) are utilized, with datasets from the Cleveland and IEEE Dataport. Optimizing model accuracy, GridsearchCV, and five-fold cross-validation are employed. In the Cleveland dataset, logistic regression surpassed others with 90.16% accuracy, while AdaBoost excelled in the IEEE Dataport dataset, achieving 90% accuracy. A soft voting ensemble classifier combining all six algorithms further enhanced accuracy, resulting in a 93.44% accuracy for the Cleveland dataset and 95% for the IEEE Dataport dataset. This surpassed the performance of the logistic regression and AdaBoost classifiers on both datasets. This study’s novelty lies in the use of GridSearchCV with five-fold cross-validation for hyperparameter optimization, determining the best parameters for the model, and assessing performance using accuracy and negative log loss metrics. This study also examined accuracy loss for each fold to evaluate the model’s performance on both benchmark datasets. The soft voting ensemble classifier approach improved accuracies on both datasets and, when compared to existing heart disease prediction studies, this method notably exceeded their results.
Record ID
Keywords
heart disease prediction, Machine Learning, performance matrices, soft voting ensemble classifier
Subject
Suggested Citation
Chandrasekhar N, Peddakrishna S. Enhancing Heart Disease Prediction Accuracy through Machine Learning Techniques and Optimization. (2023). LAPSE:2023.35283
Author Affiliations
Journal Name
Processes
Volume
11
Issue
4
First Page
1210
Year
2023
Publication Date
2023-04-14
Published Version
ISSN
2227-9717
Version Comments
Original Submission
Other Meta
PII: pr11041210, Publication Type: Journal Article
Record Map
Published Article
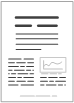
LAPSE:2023.35283
This Record
External Link
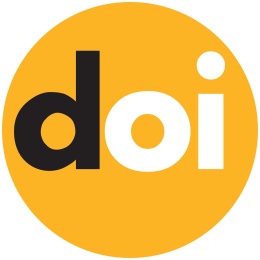
doi:10.3390/pr11041210
Publisher Version
Download
Meta
Record Statistics
Record Views
133
Version History
[v1] (Original Submission)
Apr 28, 2023
Verified by curator on
Apr 28, 2023
This Version Number
v1
Citations
Most Recent
This Version
URL Here
https://psecommunity.org/LAPSE:2023.35283
Original Submitter
Auto Uploader for LAPSE
Links to Related Works