LAPSE:2023.35282
Published Article
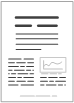
LAPSE:2023.35282
Surface Crack Detection of Steel Structures in Railroad Industry Based on Multi-Model Training Comparison Technique
April 28, 2023
A method of steel structure surface crack identification based on artificial intelligence technology is proposed to solve the problem that steel cracks can not be detected and forewarned in time when they appear in the railway industrial environment. The appearance of steel cracks greatly weakens the stability of steel structures, and will seriously endanger the safety of the railway industry if it is not detected and repaired in time. However, the common steel crack detection methods cannot achieve real-time monitoring of steel structures. In order to monitor the surface of steel structure in real-time and explore the recognition effect and model the advantages of common classification neural network models for surface cracks of railway industrial steel, this study evaluates the network model with multiple indicators and parameters under two experimental conditions. In this study, the steel surface cracks in the railway industrial environment are taken as samples, and the steel cracks are identified through the neural network model. For large-volume datasets, the recognition accuracy of the three network models has reached 97%, of which the YOLOv5 model has the best comprehensive recognition ability, and the C-Alex model has the best performance and convergence speed in small-volume datasets. This study explores the application prospects of models under different scenarios, proving that the three models can effectively detect steel surface cracks in real-time, and at the same time, it will pave the way for the development and application of artificial intelligence multi-model fusion technology in the field of the railway industry.
Record ID
Keywords
computer vision, DCNN, railway industry, steel crack identification and detection
Subject
Suggested Citation
Chen K, Huang Z, Chen C, Cheng Y, Shang Y, Zhu P, Jv H, Li L, Li W, Wang S. Surface Crack Detection of Steel Structures in Railroad Industry Based on Multi-Model Training Comparison Technique. (2023). LAPSE:2023.35282
Author Affiliations
Chen K: Department of Information Engineering, Nanjing University of Aeronautics and Astronautics Jincheng College, Nanjing 211156, China
Huang Z: Department of Information Engineering, Nanjing University of Aeronautics and Astronautics Jincheng College, Nanjing 211156, China
Chen C: Department of Mechanical and Electrical Engineering and Automation, Nanjing University of Aeronautics and Astronautics Jincheng College, Nanjing 211156, China
Cheng Y: Department of Information Engineering, Nanjing University of Aeronautics and Astronautics Jincheng College, Nanjing 211156, China
Shang Y: Department of Geography and Bioinformatics, Nanjing University of Posts and Telecommunications, Nanjing 210023, China
Zhu P: Department of Information Engineering, Nanjing University of Aeronautics and Astronautics Jincheng College, Nanjing 211156, China
Jv H: Department of Information Engineering, Nanjing University of Aeronautics and Astronautics Jincheng College, Nanjing 211156, China
Li L: Department of Information Engineering, Nanjing University of Aeronautics and Astronautics Jincheng College, Nanjing 211156, China
Li W: Department of Mechanical and Electrical Engineering and Automation, Nanjing University of Aeronautics and Astronautics Jincheng College, Nanjing 211156, China
Wang S: Department of Information Engineering, Nanjing University of Aeronautics and Astronautics Jincheng College, Nanjing 211156, China
Huang Z: Department of Information Engineering, Nanjing University of Aeronautics and Astronautics Jincheng College, Nanjing 211156, China
Chen C: Department of Mechanical and Electrical Engineering and Automation, Nanjing University of Aeronautics and Astronautics Jincheng College, Nanjing 211156, China
Cheng Y: Department of Information Engineering, Nanjing University of Aeronautics and Astronautics Jincheng College, Nanjing 211156, China
Shang Y: Department of Geography and Bioinformatics, Nanjing University of Posts and Telecommunications, Nanjing 210023, China
Zhu P: Department of Information Engineering, Nanjing University of Aeronautics and Astronautics Jincheng College, Nanjing 211156, China
Jv H: Department of Information Engineering, Nanjing University of Aeronautics and Astronautics Jincheng College, Nanjing 211156, China
Li L: Department of Information Engineering, Nanjing University of Aeronautics and Astronautics Jincheng College, Nanjing 211156, China
Li W: Department of Mechanical and Electrical Engineering and Automation, Nanjing University of Aeronautics and Astronautics Jincheng College, Nanjing 211156, China
Wang S: Department of Information Engineering, Nanjing University of Aeronautics and Astronautics Jincheng College, Nanjing 211156, China
Journal Name
Processes
Volume
11
Issue
4
First Page
1208
Year
2023
Publication Date
2023-04-14
Published Version
ISSN
2227-9717
Version Comments
Original Submission
Other Meta
PII: pr11041208, Publication Type: Journal Article
Record Map
Published Article
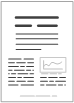
LAPSE:2023.35282
This Record
External Link
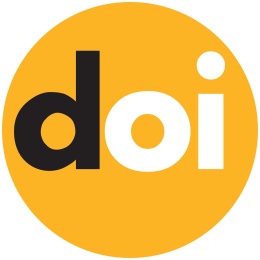
doi:10.3390/pr11041208
Publisher Version
Download
Meta
Record Statistics
Record Views
122
Version History
[v1] (Original Submission)
Apr 28, 2023
Verified by curator on
Apr 28, 2023
This Version Number
v1
Citations
Most Recent
This Version
URL Here
https://psecommunity.org/LAPSE:2023.35282
Original Submitter
Auto Uploader for LAPSE
Links to Related Works