LAPSE:2023.35113
Published Article
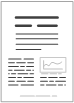
LAPSE:2023.35113
GCCSwin-UNet: Global Context and Cross-Shaped Windows Vision Transformer Network for Polyp Segmentation
April 28, 2023
Accurate polyp segmentation is of great importance for the diagnosis and treatment of colon cancer. Convolutional neural networks (CNNs) have made significant strides in the processing of medical images in recent years. The limited structure of convolutional operations prevents CNNs from learning adequately about global and long-range semantic information interactions, despite the remarkable performance they have attained. Therefore, the GCCSwin-UNet framework is suggested in this study. Specifically, the model utilizes an encoder−decoder structure, using the patch-embedding layer for feature downsampling and the CSwin Transformer block as the encoder for contextual feature extraction. To restore the feature map’s spatial resolution during upsampling operations, a symmetric decoder and patch expansion layer are also created. In order to help the backbone module to do better feature learning, we also create a global context module (GCM) and a local position-enhanced module (LPEM). We conducted extensive experiments on the Kvasir-SEG and CVC-ClinicDB datasets, and compared them with existing methods. GCCSwin-UNet reached remarkable results with Dice and MIoU of 86.37% and 83.19% for Kvasir-SEG, respectively, and 91.26% and 84.65% for CVC-ClinicDB, respectively. Finally, quantitative analysis and statistical tests are applied to further demonstrate the validity and plausibility of our method.
Record ID
Keywords
colonoscopy images, colorectal cancer, deep learning, medical image segmentation, vision transformer
Subject
Suggested Citation
Zhu J, Ge M, Chang Z, Dong W. GCCSwin-UNet: Global Context and Cross-Shaped Windows Vision Transformer Network for Polyp Segmentation. (2023). LAPSE:2023.35113
Author Affiliations
Zhu J: School of Intelligence and Information Engineering, Shandong University of Traditional Chinese Medicine, Jinan 250355, China; Suzhou Institute of Biomedical Engineering and Technology, Chinese Academy of Sciences, Suzhou 215163, China
Ge M: Suzhou Institute of Biomedical Engineering and Technology, Chinese Academy of Sciences, Suzhou 215163, China
Chang Z: Suzhou Institute of Biomedical Engineering and Technology, Chinese Academy of Sciences, Suzhou 215163, China
Dong W: School of Intelligence and Information Engineering, Shandong University of Traditional Chinese Medicine, Jinan 250355, China; Suzhou Institute of Biomedical Engineering and Technology, Chinese Academy of Sciences, Suzhou 215163, China [ORCID]
Ge M: Suzhou Institute of Biomedical Engineering and Technology, Chinese Academy of Sciences, Suzhou 215163, China
Chang Z: Suzhou Institute of Biomedical Engineering and Technology, Chinese Academy of Sciences, Suzhou 215163, China
Dong W: School of Intelligence and Information Engineering, Shandong University of Traditional Chinese Medicine, Jinan 250355, China; Suzhou Institute of Biomedical Engineering and Technology, Chinese Academy of Sciences, Suzhou 215163, China [ORCID]
Journal Name
Processes
Volume
11
Issue
4
First Page
1035
Year
2023
Publication Date
2023-03-29
Published Version
ISSN
2227-9717
Version Comments
Original Submission
Other Meta
PII: pr11041035, Publication Type: Journal Article
Record Map
Published Article
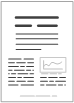
LAPSE:2023.35113
This Record
External Link
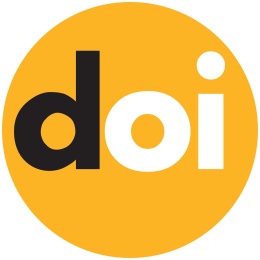
doi:10.3390/pr11041035
Publisher Version
Download
Meta
Record Statistics
Record Views
96
Version History
[v1] (Original Submission)
Apr 28, 2023
Verified by curator on
Apr 28, 2023
This Version Number
v1
Citations
Most Recent
This Version
URL Here
https://psecommunity.org/LAPSE:2023.35113
Original Submitter
Auto Uploader for LAPSE
Links to Related Works