LAPSE:2023.35068
Published Article
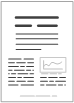
LAPSE:2023.35068
Prediction of Oxygen Content in Boiler Flue Gas Based on a Convolutional Neural Network
April 28, 2023
As one of the core pieces of equipment of the thermal power generation system, the economic and environmental performance of a boiler determines the energy efficiency of the thermal power generation unit. The oxygen content in boiler flue gas is an important parameter reflecting the combustion status of the furnace, and accurate prediction of flue gas oxygen content is of great significance for online boiler optimization. In order to solve the online prediction problem of the oxygen content in boiler flue gas, a CNN is applied to build a time series prediction model, which takes the time series samples within a fixed time window as the input of the model and uses several feature extraction modules containing convolutional, activation, and pooling layers for feature extraction and compression, and the model output is the oxygen content in boiler flue gas. Since the oxygen content in boiler flue gas is not only correlated with other variables but also influenced by its own historical trend, the input of the CNN model is improved, and an oxygen content in boiler flue gas time series prediction model (TS-CNN) is established, which takes the historical values of the boiler flue gas oxygen content as the input of the model. The comparison test results show that the R2 and RMSE of the TS-CNN model are 0.8929 and 0.1684, respectively. The prediction accuracy is higher than the CNN model, LSSVM model, and BPNN model by 18.6%, 31.2%, and 54.6%, respectively.
Record ID
Keywords
convolutional neural network, feature extraction, online prediction, oxygen content in boiler flue gas
Suggested Citation
Li Z, Li G, Shi B. Prediction of Oxygen Content in Boiler Flue Gas Based on a Convolutional Neural Network. (2023). LAPSE:2023.35068
Author Affiliations
Li Z: School of Chemistry, Chemical Engineering and Life Sciences, Wuhan University of Technology, Wuhan 430070, China
Li G: School of Chemistry, Chemical Engineering and Life Sciences, Wuhan University of Technology, Wuhan 430070, China
Shi B: School of Chemistry, Chemical Engineering and Life Sciences, Wuhan University of Technology, Wuhan 430070, China
Li G: School of Chemistry, Chemical Engineering and Life Sciences, Wuhan University of Technology, Wuhan 430070, China
Shi B: School of Chemistry, Chemical Engineering and Life Sciences, Wuhan University of Technology, Wuhan 430070, China
Journal Name
Processes
Volume
11
Issue
4
First Page
990
Year
2023
Publication Date
2023-03-24
Published Version
ISSN
2227-9717
Version Comments
Original Submission
Other Meta
PII: pr11040990, Publication Type: Journal Article
Record Map
Published Article
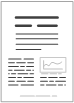
LAPSE:2023.35068
This Record
External Link
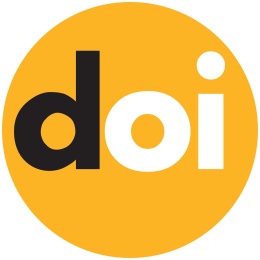
doi:10.3390/pr11040990
Publisher Version
Download
Meta
Record Statistics
Record Views
89
Version History
[v1] (Original Submission)
Apr 28, 2023
Verified by curator on
Apr 28, 2023
This Version Number
v1
Citations
Most Recent
This Version
URL Here
https://psecommunity.org/LAPSE:2023.35068
Original Submitter
Auto Uploader for LAPSE
Links to Related Works