LAPSE:2023.35023
Published Article
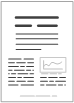
LAPSE:2023.35023
Machine-Learning-Based Classification for Pipeline Corrosion with Monte Carlo Probabilistic Analysis
April 28, 2023
Pipeline corrosion is one of the leading causes of failures in the transmission of gas and hazardous liquids in the oil and gas industry. In-line inspection is a non-destructive inspection for detecting corrosion defects in pipelines. Defects are measured in terms of their width, length and depth. Consecutive in-line inspection data are used to determine the pipeline’s corrosion growth rate and its remnant life, which set the operational and maintenance activities of the pipeline. The traditional approach of manually processing in-line inspection data has various weaknesses, including being time consuming due to huge data volume and complexity, prone to error, subject to biased judgement by experts and challenging for matching of in-line inspection datasets. This paper aimed to contribute to the adoption of machine learning approaches in classifying pipeline defects as per Pipeline Operator Forum requirements and matching in-line inspection data for determining the corrosion growth rate and remnant life of pipelines. Machine learning techniques, namely, decision tree, random forest, support vector machines and logistic regression, were applied in the classification of pipeline defects using Phyton programming. The performance of each technique in terms of the accuracy of results was compared. The results showed that the decision tree classifier model was the most accurate (99.9%) compared with the other classifiers.
Record ID
Keywords
in-line inspection, Machine Learning, pipeline corrosion, reliability analysis
Subject
Suggested Citation
Ismail MFH, May Z, Asirvadam VS, Nayan NA. Machine-Learning-Based Classification for Pipeline Corrosion with Monte Carlo Probabilistic Analysis. (2023). LAPSE:2023.35023
Author Affiliations
Ismail MFH: Electrical and Electronic Engineering Department, Universiti Teknologi PETRONAS, Seri Iskandar 32610, Perak, Malaysia [ORCID]
May Z: Electrical and Electronic Engineering Department, Universiti Teknologi PETRONAS, Seri Iskandar 32610, Perak, Malaysia; Department of Electrical, Electronic and Systems Engineering, Faculty of Engineering and Built Environment, Universiti Kebangsaan Malays
Asirvadam VS: Electrical and Electronic Engineering Department, Universiti Teknologi PETRONAS, Seri Iskandar 32610, Perak, Malaysia [ORCID]
Nayan NA: Department of Electrical, Electronic and Systems Engineering, Faculty of Engineering and Built Environment, Universiti Kebangsaan Malaysia, Bangi 43600, Selangor, Malaysia; Institute Islam Hadhari, Universiti Kebangsaan Malaysia, Bangi 43600, Selangor, Ma [ORCID]
May Z: Electrical and Electronic Engineering Department, Universiti Teknologi PETRONAS, Seri Iskandar 32610, Perak, Malaysia; Department of Electrical, Electronic and Systems Engineering, Faculty of Engineering and Built Environment, Universiti Kebangsaan Malays
Asirvadam VS: Electrical and Electronic Engineering Department, Universiti Teknologi PETRONAS, Seri Iskandar 32610, Perak, Malaysia [ORCID]
Nayan NA: Department of Electrical, Electronic and Systems Engineering, Faculty of Engineering and Built Environment, Universiti Kebangsaan Malaysia, Bangi 43600, Selangor, Malaysia; Institute Islam Hadhari, Universiti Kebangsaan Malaysia, Bangi 43600, Selangor, Ma [ORCID]
Journal Name
Energies
Volume
16
Issue
8
First Page
3589
Year
2023
Publication Date
2023-04-21
Published Version
ISSN
1996-1073
Version Comments
Original Submission
Other Meta
PII: en16083589, Publication Type: Journal Article
Record Map
Published Article
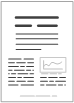
LAPSE:2023.35023
This Record
External Link
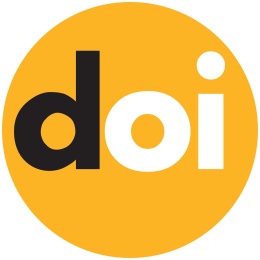
doi:10.3390/en16083589
Publisher Version
Download
Meta
Record Statistics
Record Views
97
Version History
[v1] (Original Submission)
Apr 28, 2023
Verified by curator on
Apr 28, 2023
This Version Number
v1
Citations
Most Recent
This Version
URL Here
https://psecommunity.org/LAPSE:2023.35023
Original Submitter
Auto Uploader for LAPSE
Links to Related Works