LAPSE:2023.34980
Published Article
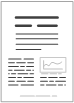
LAPSE:2023.34980
A Hybrid Deep Neural Network Architecture for Day-Ahead Electricity Forecasting: Post-COVID Paradigm
April 28, 2023
Predicting energy demand in adverse scenarios, such as the COVID-19 pandemic, is critical to ensure the supply of electricity and the operation of essential services in metropolitan regions. In this paper, we propose a deep learning model to predict the demand for the next day using the “IEEE DataPort Competition Day-Ahead Electricity Demand Forecasting: Post-COVID Paradigm” database. The best model uses hybrid deep neural network architecture (convolutional network−recurrent network) to extract spatial-temporal features from the input data. A preliminary analysis of the input data was performed, excluding anomalous variables. A sliding window was applied for importing the data into the network input. The input data was normalized, using a higher weight for the demand variable. The proposed model’s performance was better than the models that stood out in the competition, with a mean absolute error of 2361.84 kW. The high similarity between the actual demand curve and the predicted demand curve evidences the efficiency of the application of deep networks compared with the classical methods applied by other authors. In the pandemic scenario, the applied technique proved to be the best strategy to predict demand for the next day.
Record ID
Keywords
convolutional neural network, COVID-19, load forecasting, recurrent neural network
Subject
Suggested Citation
Vilaça NL, Costa MGF, Costa Filho CFF. A Hybrid Deep Neural Network Architecture for Day-Ahead Electricity Forecasting: Post-COVID Paradigm. (2023). LAPSE:2023.34980
Author Affiliations
Vilaça NL: Graduate Program in Electrical Engineering, Faculty of Technology, Federal University of Amazonas, Manaus 69077-000, Brazil [ORCID]
Costa MGF: Graduate Program in Electrical Engineering, Faculty of Technology, and R&D Center in Electronic and Information Technology, Federal University of Amazonas, Manaus 69077-000, Brazil [ORCID]
Costa Filho CFF: Graduate Program in Electrical Engineering, Faculty of Technology, and R&D Center in Electronic and Information Technology, Federal University of Amazonas, Manaus 69077-000, Brazil [ORCID]
Costa MGF: Graduate Program in Electrical Engineering, Faculty of Technology, and R&D Center in Electronic and Information Technology, Federal University of Amazonas, Manaus 69077-000, Brazil [ORCID]
Costa Filho CFF: Graduate Program in Electrical Engineering, Faculty of Technology, and R&D Center in Electronic and Information Technology, Federal University of Amazonas, Manaus 69077-000, Brazil [ORCID]
Journal Name
Energies
Volume
16
Issue
8
First Page
3546
Year
2023
Publication Date
2023-04-19
Published Version
ISSN
1996-1073
Version Comments
Original Submission
Other Meta
PII: en16083546, Publication Type: Journal Article
Record Map
Published Article
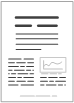
LAPSE:2023.34980
This Record
External Link
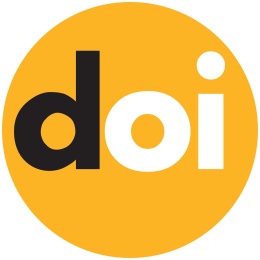
doi:10.3390/en16083546
Publisher Version
Download
Meta
Record Statistics
Record Views
79
Version History
[v1] (Original Submission)
Apr 28, 2023
Verified by curator on
Apr 28, 2023
This Version Number
v1
Citations
Most Recent
This Version
URL Here
https://psecommunity.org/LAPSE:2023.34980
Original Submitter
Auto Uploader for LAPSE
Links to Related Works