LAPSE:2023.34885
Published Article
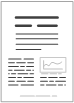
LAPSE:2023.34885
Pre-Attention Mechanism and Convolutional Neural Network Based Multivariate Load Prediction for Demand Response
April 28, 2023
The construction of smart grids has greatly changed the power grid pattern and power supply structure. For the power system, reasonable power planning and demand response is necessary to ensure the stable operation of a society. Accurate load prediction is the basis for realizing demand response for the power system. This paper proposes a Pre-Attention-CNN-GRU model (PreAttCG) which combines a convolutional neural network (CNN) and gate recurrent unit (GRU) and applies the attention mechanism in front of the whole model. The PreAttCG model accepts historical load data and more than nine other factors (including temperature, wind speed, humidity, etc.) as input. The attention layer and CNN layer effectively extract the features and weights of each factor. Load forecasting is then performed by the prediction layer, which consists of a stacked GRU. The model is verified by industrial load data from a German dataset and a Chinese dataset from the real world. The results show that the PreAttCG model has better performance (3~5% improvement in MAPE) than both LSTM with only load input and LSTM with all factors. Additionally, the experiments also show that the attention mechanism can effectively extract the weights of relevant factors affecting the load data.
Record ID
Keywords
attention, convolutional neural network, gate recurrent unit, load prediction
Suggested Citation
He Z, Lin R, Wu B, Zhao X, Zou H. Pre-Attention Mechanism and Convolutional Neural Network Based Multivariate Load Prediction for Demand Response. (2023). LAPSE:2023.34885
Author Affiliations
He Z: State Key Laboratory of Networking and Switching Technology, School of Computer Science (National Pilot Software Engineering School), Beijing University of Posts and Telecommunications, Beijing 100876, China [ORCID]
Lin R: State Key Laboratory of Networking and Switching Technology, School of Computer Science (National Pilot Software Engineering School), Beijing University of Posts and Telecommunications, Beijing 100876, China [ORCID]
Wu B: State Key Laboratory of Networking and Switching Technology, School of Computer Science (National Pilot Software Engineering School), Beijing University of Posts and Telecommunications, Beijing 100876, China
Zhao X: Economic & Research Institute, State Grid Shandong Electric Power Company, Jinan 250021, China
Zou H: State Key Laboratory of Networking and Switching Technology, School of Computer Science (National Pilot Software Engineering School), Beijing University of Posts and Telecommunications, Beijing 100876, China
Lin R: State Key Laboratory of Networking and Switching Technology, School of Computer Science (National Pilot Software Engineering School), Beijing University of Posts and Telecommunications, Beijing 100876, China [ORCID]
Wu B: State Key Laboratory of Networking and Switching Technology, School of Computer Science (National Pilot Software Engineering School), Beijing University of Posts and Telecommunications, Beijing 100876, China
Zhao X: Economic & Research Institute, State Grid Shandong Electric Power Company, Jinan 250021, China
Zou H: State Key Laboratory of Networking and Switching Technology, School of Computer Science (National Pilot Software Engineering School), Beijing University of Posts and Telecommunications, Beijing 100876, China
Journal Name
Energies
Volume
16
Issue
8
First Page
3446
Year
2023
Publication Date
2023-04-14
Published Version
ISSN
1996-1073
Version Comments
Original Submission
Other Meta
PII: en16083446, Publication Type: Journal Article
Record Map
Published Article
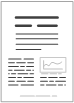
LAPSE:2023.34885
This Record
External Link
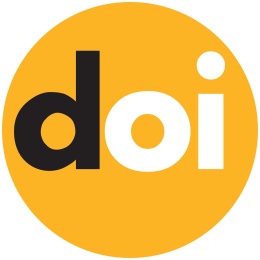
doi:10.3390/en16083446
Publisher Version
Download
Meta
Record Statistics
Record Views
95
Version History
[v1] (Original Submission)
Apr 28, 2023
Verified by curator on
Apr 28, 2023
This Version Number
v1
Citations
Most Recent
This Version
URL Here
https://psecommunity.org/LAPSE:2023.34885
Original Submitter
Auto Uploader for LAPSE
Links to Related Works