LAPSE:2023.34869
Published Article
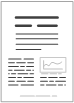
LAPSE:2023.34869
Fault Detection of Induction Motors with Combined Modeling- and Machine-Learning-Based Framework
April 28, 2023
This paper deals with the early detection of fault conditions in induction motors using a combined model- and machine-learning-based approach with flexible adaptation to individual motors. The method is based on analytical modeling in the form of a multiple coupled circuit model and a feedforward neural network. In addition, the differential evolution algorithm independently identifies the parameters of the motor for the multiple coupled circuit model based on easily obtained measurement data from a healthy state. With the identified parameters, the multiple coupled circuit model is used to perform dynamic simulations of the various fault cases of the specific induction motor. The simulation data set of the stator currents is used to train the neural network for classification of different stator, rotor, mechanical, and voltage supply faults. Finally, the combined method is successfully validated with measured data of faults in an induction motor, proving the transferability of the simulation-trained neural network to a real environment. Neglecting bearing faults, the fault cases from the validation data are classified with an accuracy of 94.81%.
Record ID
Keywords
Fault Detection, induction motors, Machine Learning, multiple coupled circuit model, parameter identification, supervised learning
Suggested Citation
Benninger M, Liebschner M, Kreischer C. Fault Detection of Induction Motors with Combined Modeling- and Machine-Learning-Based Framework. (2023). LAPSE:2023.34869
Author Affiliations
Benninger M: Faculty of Electronics and Computer Science, University of Applied Sciences Aalen, 73430 Aalen, Germany [ORCID]
Liebschner M: Faculty of Electronics and Computer Science, University of Applied Sciences Aalen, 73430 Aalen, Germany [ORCID]
Kreischer C: Chair for Electrical Machines and Drive Systems, Helmut Schmidt University, 22043 Hamburg, Germany [ORCID]
Liebschner M: Faculty of Electronics and Computer Science, University of Applied Sciences Aalen, 73430 Aalen, Germany [ORCID]
Kreischer C: Chair for Electrical Machines and Drive Systems, Helmut Schmidt University, 22043 Hamburg, Germany [ORCID]
Journal Name
Energies
Volume
16
Issue
8
First Page
3429
Year
2023
Publication Date
2023-04-13
Published Version
ISSN
1996-1073
Version Comments
Original Submission
Other Meta
PII: en16083429, Publication Type: Journal Article
Record Map
Published Article
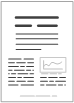
LAPSE:2023.34869
This Record
External Link
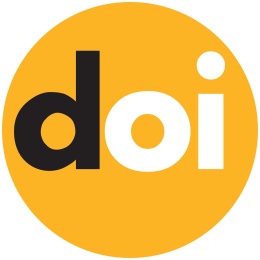
doi:10.3390/en16083429
Publisher Version
Download
Meta
Record Statistics
Record Views
145
Version History
[v1] (Original Submission)
Apr 28, 2023
Verified by curator on
Apr 28, 2023
This Version Number
v1
Citations
Most Recent
This Version
URL Here
https://psecommunity.org/LAPSE:2023.34869
Original Submitter
Auto Uploader for LAPSE
Links to Related Works