LAPSE:2023.34782
Published Article
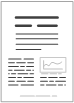
LAPSE:2023.34782
Research on Smart Power Sales Strategy Considering Load Forecasting and Optimal Allocation of Energy Storage System in China
April 28, 2023
With the deepening reform of the power system, power sales companies need to adopt new power sales strategies to provide customers with better economic marketing solutions. Customer-side configuration of an energy storage system (ESS) can participate in power-related policies to reduce the comprehensive cost of electricity for commercial and industrial customers and improve customer revenue. For power sales companies, this can also attract new customers, expand sales and quickly capture the market. However, most of the ESS evaluation models studied so far are based on historical data configuration of typical daily storage capacity and charging and discharging scheduling instructions. In addition, most models do not adequately consider the performance characteristics of the ESS and cannot accurately assess the economics of the energy storage model. This study proposes an intelligent power sales strategy based on load forecasting with the participation of optimal allocation of ESS. Based on long short-term memory (LSTM) artificial neural network for predictive analysis of customer load, we evaluate the economics of adding energy storage to customers. Based on the premise of the two-part tariff, the ESS evaluation model is constructed with the objective of minimizing the annual comprehensive cost to the user by considering the energy tariff and the savings benefits of the basic tariff, assessing the annualized cost of ESS over its entire life cycle, and the impact of battery capacity decay on economics. The particle swarm optimization (PSO) algorithm is introduced to solve the model. By simulating the arithmetic example for real customers, their integrated electricity costs are significantly reduced. Moreover, this smart power sales strategy can provide different sales strategies according to the expected payback period of customers. This smart sales strategy can output more accurate declared maximum demand values than other traditional sales strategies, providing a more economical solution for customers.
Record ID
Keywords
demand control, energy storage systems (ESS), load forecast, long short-term memory (LSTM), particle swarm optimization (PSO), peak-valley electricity arbitrage, smart power sales
Subject
Suggested Citation
Liu H, Wang L, Li J, Shao L, Zhang D. Research on Smart Power Sales Strategy Considering Load Forecasting and Optimal Allocation of Energy Storage System in China. (2023). LAPSE:2023.34782
Author Affiliations
Liu H: Tianjin Key Laboratory of New Energy Power Conversion, Transmission and Intelligent Control, Tianjin University of Technology, Tianjin 300384, China
Wang L: Tianjin Key Laboratory of New Energy Power Conversion, Transmission and Intelligent Control, Tianjin University of Technology, Tianjin 300384, China; School of Electrical Engineering and Automation, Tianjin University of Technology, Tianjin 300384, China [ORCID]
Li J: Tianjin Key Laboratory of New Energy Power Conversion, Transmission and Intelligent Control, Tianjin University of Technology, Tianjin 300384, China [ORCID]
Shao L: Tianjin Key Laboratory of New Energy Power Conversion, Transmission and Intelligent Control, Tianjin University of Technology, Tianjin 300384, China
Zhang D: Tianjin Key Laboratory of New Energy Power Conversion, Transmission and Intelligent Control, Tianjin University of Technology, Tianjin 300384, China
Wang L: Tianjin Key Laboratory of New Energy Power Conversion, Transmission and Intelligent Control, Tianjin University of Technology, Tianjin 300384, China; School of Electrical Engineering and Automation, Tianjin University of Technology, Tianjin 300384, China [ORCID]
Li J: Tianjin Key Laboratory of New Energy Power Conversion, Transmission and Intelligent Control, Tianjin University of Technology, Tianjin 300384, China [ORCID]
Shao L: Tianjin Key Laboratory of New Energy Power Conversion, Transmission and Intelligent Control, Tianjin University of Technology, Tianjin 300384, China
Zhang D: Tianjin Key Laboratory of New Energy Power Conversion, Transmission and Intelligent Control, Tianjin University of Technology, Tianjin 300384, China
Journal Name
Energies
Volume
16
Issue
8
First Page
3341
Year
2023
Publication Date
2023-04-09
Published Version
ISSN
1996-1073
Version Comments
Original Submission
Other Meta
PII: en16083341, Publication Type: Journal Article
Record Map
Published Article
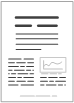
LAPSE:2023.34782
This Record
External Link
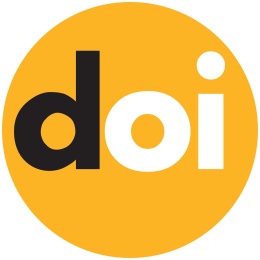
doi:10.3390/en16083341
Publisher Version
Download
Meta
Record Statistics
Record Views
92
Version History
[v1] (Original Submission)
Apr 28, 2023
Verified by curator on
Apr 28, 2023
This Version Number
v1
Citations
Most Recent
This Version
URL Here
https://psecommunity.org/LAPSE:2023.34782
Original Submitter
Auto Uploader for LAPSE
Links to Related Works