LAPSE:2023.34249
Published Article
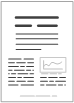
LAPSE:2023.34249
Less Information, Similar Performance: Comparing Machine Learning-Based Time Series of Wind Power Generation to Renewables.ninja
April 25, 2023
Driven by climatic processes, wind power generation is inherently variable. Long-term simulated wind power time series are therefore an essential component for understanding the temporal availability of wind power and its integration into future renewable energy systems. In the recent past, mainly power curve-based models such as Renewables.ninja (RN) have been used for deriving synthetic time series for wind power generation, despite their need for accurate location information and bias correction, as well as their insufficient replication of extreme events and short-term power ramps. In this paper, we assessed how time series generated by machine learning models (MLMs) compare to RN in terms of their ability to replicate the characteristics of observed nationally aggregated wind power generation for Germany. Hence, we applied neural networks to one wind speed input dataset derived from MERRA2 reanalysis with no location information and two with additional location information. The resulting time series and RN time series were compared with actual generation. All MLM time series feature an equal or even better time series quality than RN, depending on the characteristics considered. We conclude that MLM models show a similar performance to RN, even when information on turbine locations and turbine types is unavailable.
Record ID
Keywords
Machine Learning, reanalysis, wind power simulation, wind power time series
Subject
Suggested Citation
Baumgartner J, Gruber K, Simoes SG, Saint-Drenan YM, Schmidt J. Less Information, Similar Performance: Comparing Machine Learning-Based Time Series of Wind Power Generation to Renewables.ninja. (2023). LAPSE:2023.34249
Author Affiliations
Baumgartner J: Institute for Sustainable Economic Development, University of Natural Resources and Life Sciences, 1180 Vienna, Austria
Gruber K: Institute for Sustainable Economic Development, University of Natural Resources and Life Sciences, 1180 Vienna, Austria [ORCID]
Simoes SG: LNEG—The National Laboratory for Energy and Geology, Resource Economics Unit, 1649-038 Lisbon, Portugal
Saint-Drenan YM: MINES ParisTech, PSL Research University, O.I.E. Centre Observation, Impacts, Energy, 06904 Sophia Antipolis, France
Schmidt J: Institute for Sustainable Economic Development, University of Natural Resources and Life Sciences, 1180 Vienna, Austria
Gruber K: Institute for Sustainable Economic Development, University of Natural Resources and Life Sciences, 1180 Vienna, Austria [ORCID]
Simoes SG: LNEG—The National Laboratory for Energy and Geology, Resource Economics Unit, 1649-038 Lisbon, Portugal
Saint-Drenan YM: MINES ParisTech, PSL Research University, O.I.E. Centre Observation, Impacts, Energy, 06904 Sophia Antipolis, France
Schmidt J: Institute for Sustainable Economic Development, University of Natural Resources and Life Sciences, 1180 Vienna, Austria
Journal Name
Energies
Volume
13
Issue
9
Article Number
E2277
Year
2020
Publication Date
2020-05-05
Published Version
ISSN
1996-1073
Version Comments
Original Submission
Other Meta
PII: en13092277, Publication Type: Journal Article
Record Map
Published Article
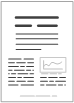
LAPSE:2023.34249
This Record
External Link
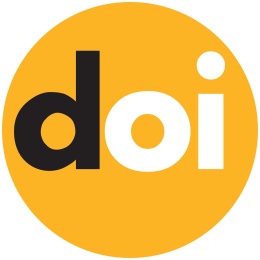
doi:10.3390/en13092277
Publisher Version
Download
Meta
Record Statistics
Record Views
83
Version History
[v1] (Original Submission)
Apr 25, 2023
Verified by curator on
Apr 25, 2023
This Version Number
v1
Citations
Most Recent
This Version
URL Here
https://psecommunity.org/LAPSE:2023.34249
Original Submitter
Auto Uploader for LAPSE
Links to Related Works