LAPSE:2023.34242
Published Article
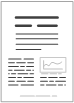
LAPSE:2023.34242
The Impact of Surrogate Models on the Multi-Objective Optimization of Pump-As-Turbine (PAT)
April 25, 2023
Pump-as-turbine (PAT) technology permits two operating states—as a pump or turbine, depending on the demand. Nevertheless, designing the geometrical components to suit these operating states has been an unending design issue, because of the multi-conditions for the PAT technology that must be attained to enhance the hydraulic performance. Also, PAT has been known to have a narrow operating range and operates poorly at off-design conditions, due to the lack of flow control device and poor geometrical designs. Therefore, for the PAT to have a wider operating range and operate effectively at off-design conditions, the geometric parameters need to be optimized. Since it is practically impossible to optimize more than one objective function at the same time, a suitable surrogate model is needed to mimic the objective functions for it to be solvable. In this study, the Latin hypercube sampling method was used to obtain the objective function values, the Adaptive Neuro-Fuzzy Inference System (ANFIS), Artificial Neural Network (ANN) and Generalized Regression Neural Network (GRNN) were used as surrogate models to approximate the objective functions in the design space. Then, a suitable surrogate model was chosen for the optimization. The Pareto-optimal solutions were obtained by using the Pareto-based genetic algorithm (PBGA). To evaluate the results of the optimization, three representative Pareto-optimal points were selected and analyzed. Compared to the baseline model, the Pareto-optimal points showed a great improvement in the objective functions. After optimization, the geometry of the impeller was redesigned to suit the operating conditions of PAT. The findings show that the efficiencies of the optimized design variables of PAT were enhanced by 23.7%, 11.5%, and 10.4% at part load, design point, and under overload flow conditions, respectively. Moreover, the results also indicated that the chosen design variables (b2, β2, β1, and z) had a substantial impact on the objective functions, justifying the feasibility of the optimization method employed in this study.
Record ID
Keywords
Adaptive Neuro-Fuzzy Inference System, General Regression Neural Network, multi-objective, Optimization, pump as turbine
Suggested Citation
Ntiri Asomani S, Yuan J, Wang L, Appiah D, Adu-Poku KA. The Impact of Surrogate Models on the Multi-Objective Optimization of Pump-As-Turbine (PAT). (2023). LAPSE:2023.34242
Author Affiliations
Ntiri Asomani S: National Research Center of Pumps, Jiangsu University, Zhenjiang 212013, China
Yuan J: National Research Center of Pumps, Jiangsu University, Zhenjiang 212013, China; Institute of Fluid Engineering Equipment, JITRI, Jiangsu University, Zhenjiang 212013, China
Wang L: National Research Center of Pumps, Jiangsu University, Zhenjiang 212013, China; School of Chemistry, Physics and Mechanical Engineering, Queensland University of Technology, Brisbane 4001, Australia
Appiah D: National Research Center of Pumps, Jiangsu University, Zhenjiang 212013, China [ORCID]
Adu-Poku KA: National Research Center of Pumps, Jiangsu University, Zhenjiang 212013, China
Yuan J: National Research Center of Pumps, Jiangsu University, Zhenjiang 212013, China; Institute of Fluid Engineering Equipment, JITRI, Jiangsu University, Zhenjiang 212013, China
Wang L: National Research Center of Pumps, Jiangsu University, Zhenjiang 212013, China; School of Chemistry, Physics and Mechanical Engineering, Queensland University of Technology, Brisbane 4001, Australia
Appiah D: National Research Center of Pumps, Jiangsu University, Zhenjiang 212013, China [ORCID]
Adu-Poku KA: National Research Center of Pumps, Jiangsu University, Zhenjiang 212013, China
Journal Name
Energies
Volume
13
Issue
9
Article Number
E2271
Year
2020
Publication Date
2020-05-04
Published Version
ISSN
1996-1073
Version Comments
Original Submission
Other Meta
PII: en13092271, Publication Type: Journal Article
Record Map
Published Article
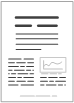
LAPSE:2023.34242
This Record
External Link
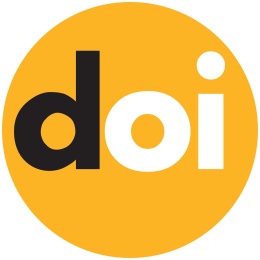
doi:10.3390/en13092271
Publisher Version
Download
Meta
Record Statistics
Record Views
100
Version History
[v1] (Original Submission)
Apr 25, 2023
Verified by curator on
Apr 25, 2023
This Version Number
v1
Citations
Most Recent
This Version
URL Here
https://psecommunity.org/LAPSE:2023.34242
Original Submitter
Auto Uploader for LAPSE
Links to Related Works