LAPSE:2023.34236
Published Article
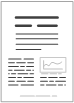
LAPSE:2023.34236
Assessment of Wind Turbine Aero-Hydro-Servo-Elastic Modelling on the Effects of Mooring Line Tension via Deep Learning
April 25, 2023
As offshore wind turbines are moving to deeper water depths, mooring systems are becoming more and more significant for floating offshore wind turbines (FOWTs). Mooring line failures could affect power generations of FOWTs and ultimately incur risk to nearby structures. Among different failure mechanics, an excessive mooring line tension is one of the most essential factors contributing to mooring failure. Even advanced sensing offers an effective way of failure detections, but it is still difficult to comprehend why failures happened. Unlike traditional parametric studies that are computational and time-intensive, this paper applies deep learning to investigate the major driven force on the mooring line tension. A number of environmental conditions are considered, ranging from cut in to cut out wind speeds. Before formatting input data into the deep learning model, a FOWT model of dynamics was simulated under pre-defined environmental conditions. Both taut and slack mooring configurations were considered in the current study. Results showed that the most loaded mooring line tension was mainly determined by the surge motion, regardless of mooring line configurations, while the blade and the tower elasticity were less significant in predicting mooring line tension.
Record ID
Keywords
deep learning, FOWT, mooring line tension
Subject
Suggested Citation
Lin Z, Liu X. Assessment of Wind Turbine Aero-Hydro-Servo-Elastic Modelling on the Effects of Mooring Line Tension via Deep Learning. (2023). LAPSE:2023.34236
Author Affiliations
Lin Z: Department of Naval Architecture, Ocean and Marine Engineering, University of Strathclyde, Glasgow G4 0LZ, UK
Liu X: James Watt School of Engineering, University of Glasgow, Glasgow G12 8QQ, UK [ORCID]
Liu X: James Watt School of Engineering, University of Glasgow, Glasgow G12 8QQ, UK [ORCID]
Journal Name
Energies
Volume
13
Issue
9
Article Number
E2264
Year
2020
Publication Date
2020-05-04
Published Version
ISSN
1996-1073
Version Comments
Original Submission
Other Meta
PII: en13092264, Publication Type: Journal Article
Record Map
Published Article
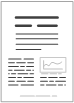
LAPSE:2023.34236
This Record
External Link
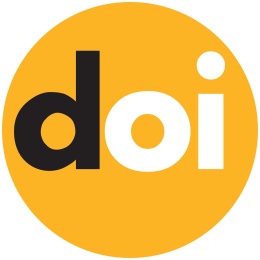
doi:10.3390/en13092264
Publisher Version
Download
Meta
Record Statistics
Record Views
86
Version History
[v1] (Original Submission)
Apr 25, 2023
Verified by curator on
Apr 25, 2023
This Version Number
v1
Citations
Most Recent
This Version
URL Here
https://psecommunity.org/LAPSE:2023.34236
Original Submitter
Auto Uploader for LAPSE
Links to Related Works