LAPSE:2023.33825
Published Article
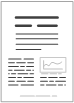
LAPSE:2023.33825
Machine Learning-Based Fault Detection and Diagnosis of Faulty Power Connections of Induction Machines
April 24, 2023
Induction machines have been key components in the industrial sector for decades, owing to different characteristics such as their simplicity, robustness, high energy efficiency and reliability. However, due to the stress and harsh working conditions they are subjected to in many applications, they are prone to suffering different breakdowns. Among the most common failure modes, bearing failures and stator winding failures can be found. To a lesser extent, High Resistance Connections (HRC) have also been investigated. Motor power connection failure mechanisms may be due to human errors while assembling the different parts of the system. Moreover, they are not only limited to HRC, there may also be cases of opposite wiring connections or open-phase faults in motor power terminals. Because of that, companies in industry are interested in diagnosing these failure modes in order to overcome human errors. This article presents a machine learning (ML) based fault diagnosis strategy to help maintenance assistants on identifying faults in the power connections of induction machines. Specifically, a strategy for failure modes such as high resistance connections, single phasing faults and opposite wiring connections has been designed. In this case, as field data under the aforementioned faulty events are scarce in industry, a simulation-driven ML-based fault diagnosis strategy has been implemented. Hence, training data for the ML algorithm has been generated via Software-in-the-Loop simulations, to train the machine learning models.
Record ID
Keywords
data-driven, electric machine, Fault Detection, fault diagnosis, induction motor, Machine Learning, power connection failures, supervised learning
Subject
Suggested Citation
Gonzalez-Jimenez D, del-Olmo J, Poza J, Garramiola F, Sarasola I. Machine Learning-Based Fault Detection and Diagnosis of Faulty Power Connections of Induction Machines. (2023). LAPSE:2023.33825
Author Affiliations
Gonzalez-Jimenez D: Faculty of Engineering, Mondragon Unibertsitatea, 20500 Arrasate-Mondragón, Gipuzkoa, Spain [ORCID]
del-Olmo J: Faculty of Engineering, Mondragon Unibertsitatea, 20500 Arrasate-Mondragón, Gipuzkoa, Spain [ORCID]
Poza J: Faculty of Engineering, Mondragon Unibertsitatea, 20500 Arrasate-Mondragón, Gipuzkoa, Spain [ORCID]
Garramiola F: Faculty of Engineering, Mondragon Unibertsitatea, 20500 Arrasate-Mondragón, Gipuzkoa, Spain [ORCID]
Sarasola I: Faculty of Engineering, Mondragon Unibertsitatea, 20500 Arrasate-Mondragón, Gipuzkoa, Spain
del-Olmo J: Faculty of Engineering, Mondragon Unibertsitatea, 20500 Arrasate-Mondragón, Gipuzkoa, Spain [ORCID]
Poza J: Faculty of Engineering, Mondragon Unibertsitatea, 20500 Arrasate-Mondragón, Gipuzkoa, Spain [ORCID]
Garramiola F: Faculty of Engineering, Mondragon Unibertsitatea, 20500 Arrasate-Mondragón, Gipuzkoa, Spain [ORCID]
Sarasola I: Faculty of Engineering, Mondragon Unibertsitatea, 20500 Arrasate-Mondragón, Gipuzkoa, Spain
Journal Name
Energies
Volume
14
Issue
16
First Page
4886
Year
2021
Publication Date
2021-08-10
Published Version
ISSN
1996-1073
Version Comments
Original Submission
Other Meta
PII: en14164886, Publication Type: Journal Article
Record Map
Published Article
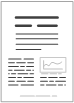
LAPSE:2023.33825
This Record
External Link
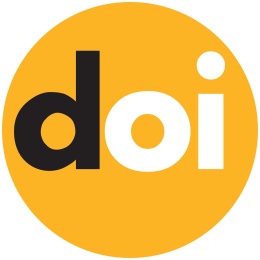
doi:10.3390/en14164886
Publisher Version
Download
Meta
Record Statistics
Record Views
137
Version History
[v1] (Original Submission)
Apr 24, 2023
Verified by curator on
Apr 24, 2023
This Version Number
v1
Citations
Most Recent
This Version
URL Here
https://psecommunity.org/LAPSE:2023.33825
Original Submitter
Auto Uploader for LAPSE
Links to Related Works