LAPSE:2023.33784
Published Article
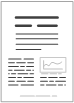
LAPSE:2023.33784
An Improved Method Based on Deep Learning for Insulator Fault Detection in Diverse Aerial Images
April 24, 2023
Insulators play a significant role in high-voltage transmission lines, and detecting insulator faults timely and accurately is important for the safe and stable operation of power grids. Since insulator faults are extremely small and the backgrounds of aerial images are complex, insulator fault detection is a challenging task for automatically inspecting transmission lines. In this paper, a method based on deep learning is proposed for insulator fault detection in diverse aerial images. Firstly, to provide sufficient insulator fault images for training, a novel insulator fault dataset named “InSF-detection” is constructed. Secondly, an improved YOLOv3 model is proposed to reuse features and prevent feature loss. To improve the accuracy of insulator fault detection, SPP-networks and a multi-scale prediction network are employed for the improved YOLOv3 model. Finally, the improved YOLOv3 model and the compared models are trained and tested on the “InSF-detection”. The average precision (AP) of the improved YOLOv3 model is superior to YOLOv3 and YOLOv3-dense models, and just a little (1.2%) lower than that of CSPD-YOLO model; more importantly, the memory usage of the improved YOLOv3 model is 225 MB, which is the smallest between the four compared models. The experimental results and analysis validate that the improved YOLOv3 model achieves good performance for insulator fault detection in aerial images with diverse backgrounds.
Record ID
Keywords
aerial image, complex backgrounds, deep learning, DenseNet, insulator fault detection, YOLO
Subject
Suggested Citation
Liu J, Liu C, Wu Y, Xu H, Sun Z. An Improved Method Based on Deep Learning for Insulator Fault Detection in Diverse Aerial Images. (2023). LAPSE:2023.33784
Author Affiliations
Liu J: College of Mechanical and Electrical Engineering, Chizhou University, Chizhou 247000, China
Liu C: College of Mechanical and Electrical Engineering, Chizhou University, Chizhou 247000, China; College of Electronic and Information Engineering, Nanjing University of Aeronautics and Astronautics, Nanjing 211106, China [ORCID]
Wu Y: College of Electronic and Information Engineering, Nanjing University of Aeronautics and Astronautics, Nanjing 211106, China
Xu H: College of Mechanical and Electrical Engineering, Chizhou University, Chizhou 247000, China
Sun Z: College of Mechanical and Electrical Engineering, Chizhou University, Chizhou 247000, China
Liu C: College of Mechanical and Electrical Engineering, Chizhou University, Chizhou 247000, China; College of Electronic and Information Engineering, Nanjing University of Aeronautics and Astronautics, Nanjing 211106, China [ORCID]
Wu Y: College of Electronic and Information Engineering, Nanjing University of Aeronautics and Astronautics, Nanjing 211106, China
Xu H: College of Mechanical and Electrical Engineering, Chizhou University, Chizhou 247000, China
Sun Z: College of Mechanical and Electrical Engineering, Chizhou University, Chizhou 247000, China
Journal Name
Energies
Volume
14
Issue
14
First Page
4365
Year
2021
Publication Date
2021-07-20
Published Version
ISSN
1996-1073
Version Comments
Original Submission
Other Meta
PII: en14144365, Publication Type: Journal Article
Record Map
Published Article
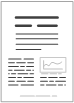
LAPSE:2023.33784
This Record
External Link
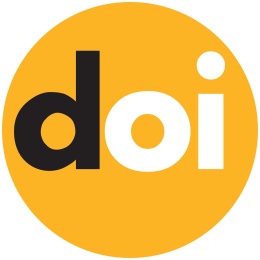
doi:10.3390/en14144365
Publisher Version
Download
Meta
Record Statistics
Record Views
83
Version History
[v1] (Original Submission)
Apr 24, 2023
Verified by curator on
Apr 24, 2023
This Version Number
v1
Citations
Most Recent
This Version
URL Here
https://psecommunity.org/LAPSE:2023.33784
Original Submitter
Auto Uploader for LAPSE
Links to Related Works