LAPSE:2023.33314
Published Article
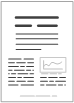
LAPSE:2023.33314
Anomaly Detection, Trend Evolution, and Feature Extraction in Partial Discharge Patterns
April 21, 2023
In the resilient and reliable electrical power system, the condition of high voltage insulation plays a crucial role. In the field of high voltage insulation integrity, the partial discharge (PD) inception and development trends are essential for assessment criteria in diagnostics systems. The observed trend to employ more and more sophisticated algorithms with machine learning features and artificial intelligence (AI) elements is observed everywhere. The classification and identification of features in PD images is perceived as a critical requirement for an effective high voltage insulation diagnosis. In this context, techniques allowing for anomaly detection, trends observation, and feature extraction in partial discharge patterns are important. In this paper, the application of few algorithms belonging to image processing, machine learning and optical flow is presented. The feature extraction refers to image segmentation and detection of coherent forms in the images. The anomaly detection algorithms can trigger early detection of the trend changes or the appearance of a new discharge form, and hence are suitable for PD monitoring applications. Anomaly detection can also handle transients and disturbances that appear in the PD image as an indication of an abnormal state. The future monitoring systems should be equipped with trend evolution algorithms. In this context, two examples of insulation aging and application of PD-based monitoring are shown. The first one refers to deep convolutional neural networks used for classification of deterioration stages in high voltage insulation. The latter one demonstrates application of optical flow approach for motion detection in partial discharge images. The motivation for the research was the strive to machine-controlled pattern analysis, leading towards intelligent PD-based diagnostics.
Record ID
Keywords
convolutional neural network, deep learning, diagnostics, high voltage insulation systems, image processing, Machine Learning, optical flow, partial discharges, phase-resolved patterns
Suggested Citation
Florkowski M. Anomaly Detection, Trend Evolution, and Feature Extraction in Partial Discharge Patterns. (2023). LAPSE:2023.33314
Author Affiliations
Florkowski M: Department of Electrical and Power Engineering, AGH University of Science and Technology, al. Mickiewicza 30, 30-059 Kraków, Poland [ORCID]
Journal Name
Energies
Volume
14
Issue
13
First Page
3886
Year
2021
Publication Date
2021-06-28
Published Version
ISSN
1996-1073
Version Comments
Original Submission
Other Meta
PII: en14133886, Publication Type: Journal Article
Record Map
Published Article
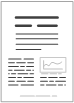
LAPSE:2023.33314
This Record
External Link
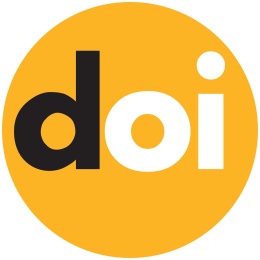
doi:10.3390/en14133886
Publisher Version
Download
Meta
Record Statistics
Record Views
75
Version History
[v1] (Original Submission)
Apr 21, 2023
Verified by curator on
Apr 21, 2023
This Version Number
v1
Citations
Most Recent
This Version
URL Here
https://psecommunity.org/LAPSE:2023.33314
Original Submitter
Auto Uploader for LAPSE
Links to Related Works