LAPSE:2023.33191
Published Article
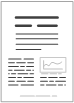
LAPSE:2023.33191
Applied Machine Learning Techniques for Performance Analysis in Large Wind Farms
April 21, 2023
As the amount of information collected by wind turbines continues to grow, so too does the potential of its leveraging. The application of machine learning techniques as an advanced analytic tool has proven effective in solving tasks whose inherent complexity can outreach expert-based ability. Such is the case presented by this study, in which the dataset to be leveraged is high-dimensional (79 turbines × 7 SCADA channels) and high-frequency (1 Hz). In this paper, a series of machine learning techniques is applied to the retrospective power performance analysis of a withheld test set containing SCADA data collectively representing 2 full days worth of operation at the Horns Rev I offshore wind farm. A sequential machine-learning based methodology is thoroughly explored, refined, then applied to the power performance analysis task of identifying instances of abnormal behaviour; namely instances of wind turbine under and over-performance. The results of the final analysis suggest that a normal behaviour model (NBM), consisting of a uniquely constructed artificial neural network (ANN) variant trained on abnormality filtered dataset, indeed proves effective in accomplishing the power performance analysis objective. Instances of over and under performance captured by the developed NBM network are presented and discussed, including the operation status of the turbines and the uncertainty embedded in the prediction results.
Record ID
Keywords
artificial neural networks, long short-term memory, Machine Learning, performance monitoring, wind farm operation and monitoring, wind farm power curve
Suggested Citation
Lyons JT, Göçmen T. Applied Machine Learning Techniques for Performance Analysis in Large Wind Farms. (2023). LAPSE:2023.33191
Author Affiliations
Lyons JT: DTU Wind Energy, Technical University of Denmark, 4000 Roskilde, Denmark
Göçmen T: DTU Wind Energy, Technical University of Denmark, 4000 Roskilde, Denmark [ORCID]
Göçmen T: DTU Wind Energy, Technical University of Denmark, 4000 Roskilde, Denmark [ORCID]
Journal Name
Energies
Volume
14
Issue
13
First Page
3756
Year
2021
Publication Date
2021-06-23
Published Version
ISSN
1996-1073
Version Comments
Original Submission
Other Meta
PII: en14133756, Publication Type: Journal Article
Record Map
Published Article
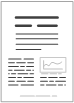
LAPSE:2023.33191
This Record
External Link
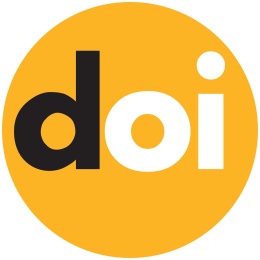
doi:10.3390/en14133756
Publisher Version
Download
Meta
Record Statistics
Record Views
74
Version History
[v1] (Original Submission)
Apr 21, 2023
Verified by curator on
Apr 21, 2023
This Version Number
v1
Citations
Most Recent
This Version
URL Here
https://psecommunity.org/LAPSE:2023.33191
Original Submitter
Auto Uploader for LAPSE
Links to Related Works