LAPSE:2023.33090
Published Article
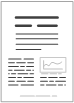
LAPSE:2023.33090
Distributed Learning Applications in Power Systems: A Review of Methods, Gaps, and Challenges
April 20, 2023
In recent years, machine learning methods have found numerous applications in power systems for load forecasting, voltage control, power quality monitoring, anomaly detection, etc. Distributed learning is a subfield of machine learning and a descendant of the multi-agent systems field. Distributed learning is a collaboratively decentralized machine learning algorithm designed to handle large data sizes, solve complex learning problems, and increase privacy. Moreover, it can reduce the risk of a single point of failure compared to fully centralized approaches and lower the bandwidth and central storage requirements. This paper introduces three existing distributed learning frameworks and reviews the applications that have been proposed for them in power systems so far. It summarizes the methods, benefits, and challenges of distributed learning frameworks in power systems and identifies the gaps in the literature for future studies.
Record ID
Keywords
assisted learning, distributed learning, federated learning, Machine Learning, power systems, privacy
Subject
Suggested Citation
Gholizadeh N, Musilek P. Distributed Learning Applications in Power Systems: A Review of Methods, Gaps, and Challenges. (2023). LAPSE:2023.33090
Author Affiliations
Gholizadeh N: Electrical and Computer Engineering, University of Alberta, Edmonton, AB T6G 1H9, Canada [ORCID]
Musilek P: Electrical and Computer Engineering, University of Alberta, Edmonton, AB T6G 1H9, Canada; Applied Cybernetics, University of Hradec Králové, 500 03 Hradec Králové, Czech Republic [ORCID]
Musilek P: Electrical and Computer Engineering, University of Alberta, Edmonton, AB T6G 1H9, Canada; Applied Cybernetics, University of Hradec Králové, 500 03 Hradec Králové, Czech Republic [ORCID]
Journal Name
Energies
Volume
14
Issue
12
First Page
3654
Year
2021
Publication Date
2021-06-19
Published Version
ISSN
1996-1073
Version Comments
Original Submission
Other Meta
PII: en14123654, Publication Type: Review
Record Map
Published Article
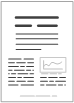
LAPSE:2023.33090
This Record
External Link
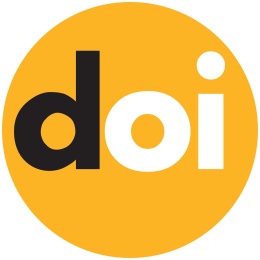
doi:10.3390/en14123654
Publisher Version
Download
Meta
Record Statistics
Record Views
77
Version History
[v1] (Original Submission)
Apr 20, 2023
Verified by curator on
Apr 20, 2023
This Version Number
v1
Citations
Most Recent
This Version
URL Here
https://psecommunity.org/LAPSE:2023.33090
Original Submitter
Auto Uploader for LAPSE
Links to Related Works