LAPSE:2023.32898
Published Article
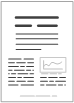
LAPSE:2023.32898
Data-Driven Energy Storage Scheduling to Minimise Peak Demand on Distribution Systems with PV Generation
April 20, 2023
The growing adoption of decentralised renewable energy generation (such as solar photovoltaic panels and wind turbines) and low-carbon technologies will increase the strain experienced by the distribution networks in the near future. In such a scenario, energy storage is becoming a key alternative to traditional expensive reinforcements to network infrastructure, due to its flexibility, decreasing costs and fast deployment capabilities. In this work, an end-to-end data-driven solution to optimally design the control of a battery unit with the aim of reducing the peak electricity demand is presented. The proposed solution uses state-of-the-art machine learning methods for forecasting electricity demand and PV generation, combined with an optimisation strategy to maximise the use of photovoltaic energy to charge the energy storage unit. To this end, historical demand, weather, and solar energy generation data collected at the Stentaway Primary substation near Plymouth, UK, and at other six locations were employed.
Record ID
Keywords
battery energy storage system, constrained optimisation under uncertainty, distribution systems, Machine Learning, photovoltaic power generation, short-term electrical load forecasting
Subject
Suggested Citation
Borghini E, Giannetti C, Flynn J, Todeschini G. Data-Driven Energy Storage Scheduling to Minimise Peak Demand on Distribution Systems with PV Generation. (2023). LAPSE:2023.32898
Author Affiliations
Borghini E: Faculty of Science and Engineering, Swansea University, Swansea SA1 8EN, UK
Giannetti C: Faculty of Science and Engineering, Swansea University, Swansea SA1 8EN, UK [ORCID]
Flynn J: Materials and Manufacturing Academy, Swansea University, Swansea SA1 8EN, UK [ORCID]
Todeschini G: Faculty of Science and Engineering, Swansea University, Swansea SA1 8EN, UK [ORCID]
Giannetti C: Faculty of Science and Engineering, Swansea University, Swansea SA1 8EN, UK [ORCID]
Flynn J: Materials and Manufacturing Academy, Swansea University, Swansea SA1 8EN, UK [ORCID]
Todeschini G: Faculty of Science and Engineering, Swansea University, Swansea SA1 8EN, UK [ORCID]
Journal Name
Energies
Volume
14
Issue
12
First Page
3453
Year
2021
Publication Date
2021-06-10
Published Version
ISSN
1996-1073
Version Comments
Original Submission
Other Meta
PII: en14123453, Publication Type: Journal Article
Record Map
Published Article
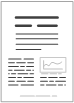
LAPSE:2023.32898
This Record
External Link
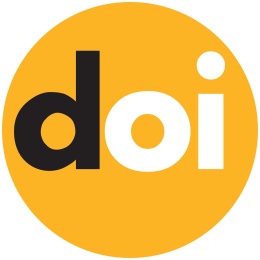
doi:10.3390/en14123453
Publisher Version
Download
Meta
Record Statistics
Record Views
75
Version History
[v1] (Original Submission)
Apr 20, 2023
Verified by curator on
Apr 20, 2023
This Version Number
v1
Citations
Most Recent
This Version
URL Here
https://psecommunity.org/LAPSE:2023.32898
Original Submitter
Auto Uploader for LAPSE
Links to Related Works