LAPSE:2023.32695
Published Article
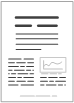
LAPSE:2023.32695
Regional Solar Irradiance Forecast for Kanto Region by Support Vector Regression Using Forecast of Meso-Ensemble Prediction System
April 20, 2023
From the perspective of stable operation of the power transmission system, the transmission system operators (TSO) needs to procure reserve adjustment power at the stage of the previous day based on solar power forecast information from global horizontal irradiance (GHI). Because the reserve adjustment power is determined based on information on major outliers in past forecasts, reducing the maximum forecast error in addition to improving the average forecast accuracy is extremely important from the perspective of grid operation. In the past, researchers have proposed various methods combining the numerical weather prediction (NWP) and machine learning techniques for the one day-ahead solar power forecasting, but the accuracy of NWP has been a bottleneck issue. In recent years, the development of the ensemble prediction system (EPS) forecasts based on probabilistic approaches has been promoted to improve the accuracy of NWP, and in Japan, EPS forecasts in the mesoscale domain, called mesoscale ensemble prediction system (MEPS), have been distributed by the Japan Meteorological Agency (JMA). The use of EPS as a machine learning model is expected to improve the maximum forecast error, as well as the accuracy, since the predictor can utilize various weather scenarios as information. The purpose of this study is to examine the effect of EPS on the GHI prediction and the structure of the machine learning model that can effectively use EPS. In this study, we constructed the support vector regression (SVR)-based predictors with multiple network configurations using MEPS as input and evaluated the forecast error of the Kanto region GHI by each model. Through the comparison of the prediction results, it was shown that the machine learning model can achieve average accuracy improvement while reducing the maximum prediction error by MEPS, and knowledge was obtained on how to effectively provide EPS information to the predictor. In addition, machine learning was found to be useful in improving the systematic error of MEPS.
Record ID
Keywords
ensemble learning, meso-ensemble prediction system, solar irradiance forecast, support vector regression
Subject
Suggested Citation
Takamatsu T, Ohtake H, Oozeki T, Nakaegawa T, Honda Y, Kazumori M. Regional Solar Irradiance Forecast for Kanto Region by Support Vector Regression Using Forecast of Meso-Ensemble Prediction System. (2023). LAPSE:2023.32695
Author Affiliations
Takamatsu T: National Institute of Advanced Industrial Science and Technology, Fukushima 963-0298, Japan [ORCID]
Ohtake H: National Institute of Advanced Industrial Science and Technology, Fukushima 963-0298, Japan; Meteorological Research Institute, Ibaraki 305-0052, Japan [ORCID]
Oozeki T: National Institute of Advanced Industrial Science and Technology, Fukushima 963-0298, Japan [ORCID]
Nakaegawa T: Meteorological Research Institute, Ibaraki 305-0052, Japan
Honda Y: Japan Meteorological Agency, Tokyo 105-8431, Japan
Kazumori M: Japan Meteorological Agency, Tokyo 105-8431, Japan
Ohtake H: National Institute of Advanced Industrial Science and Technology, Fukushima 963-0298, Japan; Meteorological Research Institute, Ibaraki 305-0052, Japan [ORCID]
Oozeki T: National Institute of Advanced Industrial Science and Technology, Fukushima 963-0298, Japan [ORCID]
Nakaegawa T: Meteorological Research Institute, Ibaraki 305-0052, Japan
Honda Y: Japan Meteorological Agency, Tokyo 105-8431, Japan
Kazumori M: Japan Meteorological Agency, Tokyo 105-8431, Japan
Journal Name
Energies
Volume
14
Issue
11
First Page
3245
Year
2021
Publication Date
2021-06-02
Published Version
ISSN
1996-1073
Version Comments
Original Submission
Other Meta
PII: en14113245, Publication Type: Journal Article
Record Map
Published Article
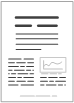
LAPSE:2023.32695
This Record
External Link
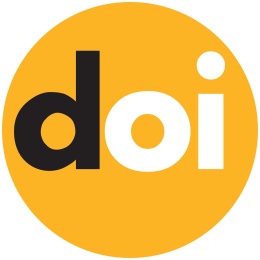
doi:10.3390/en14113245
Publisher Version
Download
Meta
Record Statistics
Record Views
67
Version History
[v1] (Original Submission)
Apr 20, 2023
Verified by curator on
Apr 20, 2023
This Version Number
v1
Citations
Most Recent
This Version
URL Here
https://psecommunity.org/LAPSE:2023.32695
Original Submitter
Auto Uploader for LAPSE
Links to Related Works