LAPSE:2023.32438
Published Article
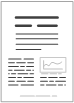
LAPSE:2023.32438
High Precision LSTM Model for Short-Time Load Forecasting in Power Systems
April 20, 2023
The paper presents the application of recurrent LSTM neural networks for short-time load forecasting in the Polish Power System (PPS) and a small region of a power system in Central Poland. The objective of the present work was to develop an efficient and accurate method of forecasting the 24-h pattern of power load with a 1-h and 24-h horizon. LSTM showed effectiveness in predicting the irregular trends in time series. The final forecast is estimated using an ensemble consisted of five independent predictions. Numerical experiments proved the superiority of the ensemble above single predictor resulting in a reduction of the MAPE the RMSE error by more than 6% in both forecasting tasks.
Record ID
Keywords
demand-side management, load forecasting, power systems, prediction systems, recurrent LSTM network
Subject
Suggested Citation
Ciechulski T, Osowski S. High Precision LSTM Model for Short-Time Load Forecasting in Power Systems. (2023). LAPSE:2023.32438
Author Affiliations
Ciechulski T: Institute of Electronic Systems, Faculty of Electronics, Military University of Technology, ul. gen. Sylwestra Kaliskiego 2, 00-908 Warsaw, Poland [ORCID]
Osowski S: Institute of Electronic Systems, Faculty of Electronics, Military University of Technology, ul. gen. Sylwestra Kaliskiego 2, 00-908 Warsaw, Poland; Faculty of Electrical Engineering, Warsaw University of Technology, pl. Politechniki 1, 00-661 Warsaw, Pola
Osowski S: Institute of Electronic Systems, Faculty of Electronics, Military University of Technology, ul. gen. Sylwestra Kaliskiego 2, 00-908 Warsaw, Poland; Faculty of Electrical Engineering, Warsaw University of Technology, pl. Politechniki 1, 00-661 Warsaw, Pola
Journal Name
Energies
Volume
14
Issue
11
First Page
2983
Year
2021
Publication Date
2021-05-21
Published Version
ISSN
1996-1073
Version Comments
Original Submission
Other Meta
PII: en14112983, Publication Type: Journal Article
Record Map
Published Article
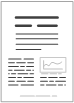
LAPSE:2023.32438
This Record
External Link
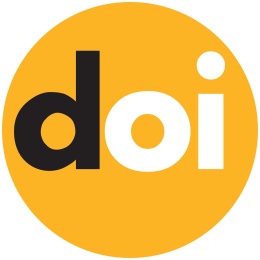
doi:10.3390/en14112983
Publisher Version
Download
Meta
Record Statistics
Record Views
73
Version History
[v1] (Original Submission)
Apr 20, 2023
Verified by curator on
Apr 20, 2023
This Version Number
v1
Citations
Most Recent
This Version
URL Here
https://psecommunity.org/LAPSE:2023.32438
Original Submitter
Auto Uploader for LAPSE
Links to Related Works