LAPSE:2023.32387
Published Article
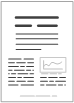
LAPSE:2023.32387
Applying Artificial Intelligence to Predict the Composition of Syngas Using Rice Husks: A Comparison of Artificial Neural Networks and Gradient Boosting Regression
April 20, 2023
The purpose of this study is to utilize two artificial intelligence (AI) models to predict the syngas composition of a fixed bed updraft gasifier for the gasification of rice husks. Air and steam-air mixtures are the gasifying agents. In the present work, the feeding rate of rice husks is kept constant, while the air and steam flow rates vary in each case. The consideration of various operating conditions provides a clear comparison between air and steam-air gasification. The effects of the reactor temperature, steam-air flow rate, and the ratio of steam to biomass are investigated here. The concentrations of combustible gases such as hydrogen, carbon monoxide, and methane in syngas are increased when using the steam-air mixture. Two AI models, namely artificial neural network (ANN) and gradient boosting regression (GBR), are applied to predict the syngas compositions using the experimental data. A total of 74 sets of data are analyzed. The compositions of five gases (CO, CO2, H2, CH4, and N2) are predicted by the ANN and GBR models. The coefficients of determination (R2) range from 0.80 to 0.89 for the ANN model, while the value of R2 ranges from 0.81 to 0.93 for GBR model. In this study, the GBR model outperforms the ANNs model based on its ensemble technique that uses multiple weak learners. As a result, the GBR model is more convincing in the prediction of syngas composition than the ANN model considered in this research.
Record ID
Keywords
artificial neural network, Biomass, gasification, gradient boosting regression, hydrogen production, rice husk, steam-air updraft gasifier
Subject
Suggested Citation
Wen HT, Lu JH, Phuc MX. Applying Artificial Intelligence to Predict the Composition of Syngas Using Rice Husks: A Comparison of Artificial Neural Networks and Gradient Boosting Regression. (2023). LAPSE:2023.32387
Author Affiliations
Wen HT: Department of Mechanical Engineering, National Chung Hsing University, Taichung City 402, Taiwan
Lu JH: Department of Mechanical Engineering, National Chung Hsing University, Taichung City 402, Taiwan
Phuc MX: Department of Mechanical Engineering, National Chung Hsing University, Taichung City 402, Taiwan
Lu JH: Department of Mechanical Engineering, National Chung Hsing University, Taichung City 402, Taiwan
Phuc MX: Department of Mechanical Engineering, National Chung Hsing University, Taichung City 402, Taiwan
Journal Name
Energies
Volume
14
Issue
10
First Page
2932
Year
2021
Publication Date
2021-05-19
Published Version
ISSN
1996-1073
Version Comments
Original Submission
Other Meta
PII: en14102932, Publication Type: Journal Article
Record Map
Published Article
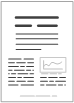
LAPSE:2023.32387
This Record
External Link
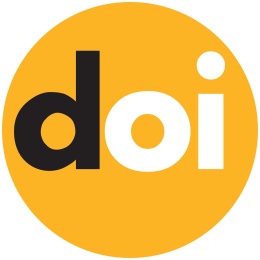
doi:10.3390/en14102932
Publisher Version
Download
Meta
Record Statistics
Record Views
78
Version History
[v1] (Original Submission)
Apr 20, 2023
Verified by curator on
Apr 20, 2023
This Version Number
v1
Citations
Most Recent
This Version
URL Here
https://psecommunity.org/LAPSE:2023.32387
Original Submitter
Auto Uploader for LAPSE
Links to Related Works