LAPSE:2023.32109
Published Article
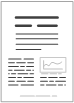
LAPSE:2023.32109
A New Model for Estimation of Bubble Point Pressure Using a Bayesian Optimized Least Square Gradient Boosting Ensemble
April 19, 2023
Accurate estimation of crude oil Bubble Point Pressure (Pb) plays a vital rule in the development cycle of an oil field. Bubble point pressure is required in many petroleum engineering calculations such as reserves estimation, material balance, reservoir simulation, production equipment design, and optimization of well performance. Additionally, bubble point pressure is a key input parameter in most oil property correlations. Thus, an error in a bubble point pressure estimate will definitely propagate additional error in the prediction of other oil properties. Accordingly, many bubble point pressure correlations have been developed in the literature. However, they often lack accuracy, especially when applied for global crude oil data, due to the fact that they are either developed using a limited range of independent variables or developed for a specific geographic location (i.e., specific crude oil composition). This research presents a utilization of the state-of-the-art Bayesian optimized Least Square Gradient Boosting Ensemble (LS-Boost) to predict bubble pointpressure as a function of readily available field data. The proposed model was trained on a global crude oil database which contains (4800) experimentally measured, Pressure−Volume−Temperature (PVT) data sets of a diverse collection of crude oil mixtures from different oil fields in the NorthSea, Africa, Asia, Middle East, and South and North America. Furthermore, an independent (775) PVT data set, which was collected from open literature, was used to investigate the effectiveness of the proposed model to predict the bubble point pressure from data that were not used during the model development process. The accuracy of the proposed model was compared to several published correlations (13 in total for both parametric and non-parametric models) as well as two other machine learning techniques, Multi-Layer Perceptron Neural Networks (MPL-ANN) and Support Vector Machines (SVM). The proposed LS-Boost model showed superior performance andremarkably outperformed all bubble point pressure models considered in this study.
Record ID
Keywords
bubble point pressure correlation, least square gradient boosting ensemble, Machine Learning
Subject
Suggested Citation
Alatefi S, Almeshal AM. A New Model for Estimation of Bubble Point Pressure Using a Bayesian Optimized Least Square Gradient Boosting Ensemble. (2023). LAPSE:2023.32109
Author Affiliations
Journal Name
Energies
Volume
14
Issue
9
First Page
2653
Year
2021
Publication Date
2021-05-05
Published Version
ISSN
1996-1073
Version Comments
Original Submission
Other Meta
PII: en14092653, Publication Type: Journal Article
Record Map
Published Article
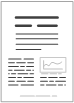
LAPSE:2023.32109
This Record
External Link
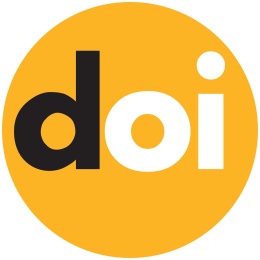
doi:10.3390/en14092653
Publisher Version
Download
Meta
Record Statistics
Record Views
86
Version History
[v1] (Original Submission)
Apr 19, 2023
Verified by curator on
Apr 19, 2023
This Version Number
v1
Citations
Most Recent
This Version
URL Here
https://psecommunity.org/LAPSE:2023.32109
Original Submitter
Auto Uploader for LAPSE
Links to Related Works